How Clear Street uses Metaplane to Prevent $100M+ Worth of Data Quality Issues
❝Metaplane is the data quality x-ray on our data stack.
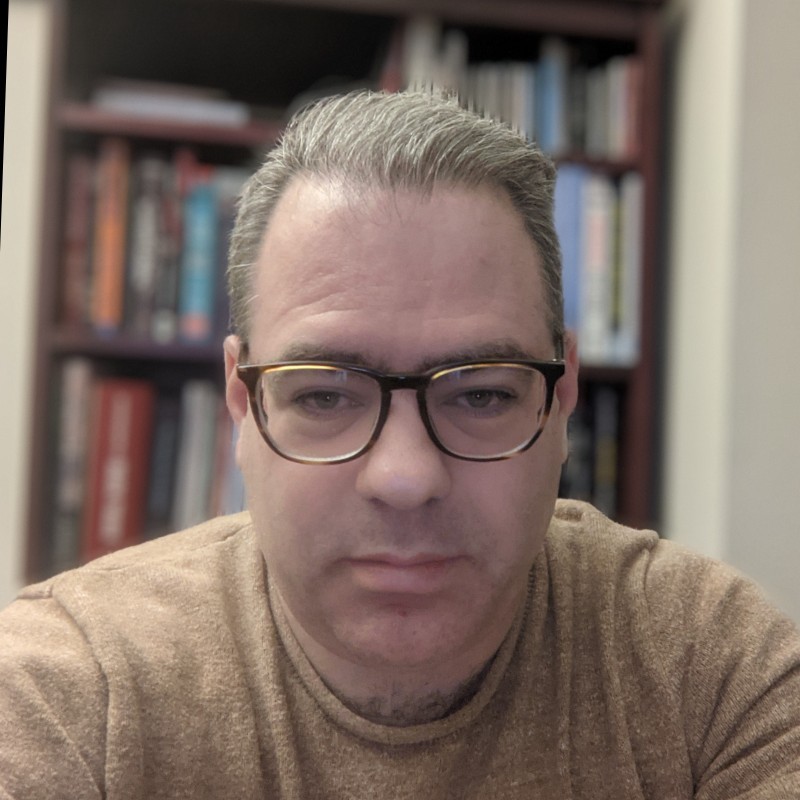
Clear Street’s prime brokerage platform is a new approach to the legacy financial infrastructure that moves trillions of dollars daily. When processing that amount of money, any small issue with a platform might be magnified into millions of dollars of loss. Clear Street’s technology-first approach allows them to treat market data at the heart of decision-making as a first-class citizen, in part by making data more accessible to financial institutions through their modern infrastructure.
That technology-first approach extends into their cloud-based modern data stack, a rarity for others in the space, that allows them to execute faster and with more certainty. We spoke with David Wasserman, Sr. Data Architect on the DATA Team, and Shawn Carroll, Sr. Developer on the FACT Team.
❝It’s a testament to Clear Street that we’re cloud-first in the staid technology landscape that is prime brokerage - we’re really ahead of the curve”
Together, the FACT and DATA team are responsible for data pipelines that power Clear Street. The FACT team ingests the referential data that helps brokers make decisions about trading decisions, risk, maintaining ledgers, and more, while the DATA Team owns analysis through business intelligence workbooks. Sigma was chosen to democratize data visualization while making insights more accessible due to its spreadsheet-like interface. Those Sigma workbooks include: financial analytics, operational statistics, and brokerage facts, on top of also managing traditional internal business analytics.
❝We’re proud of the team effort taken to move up the data maturity curve, and we’ve seen a sharp increase not only in storage, but also in usage of data across the company”
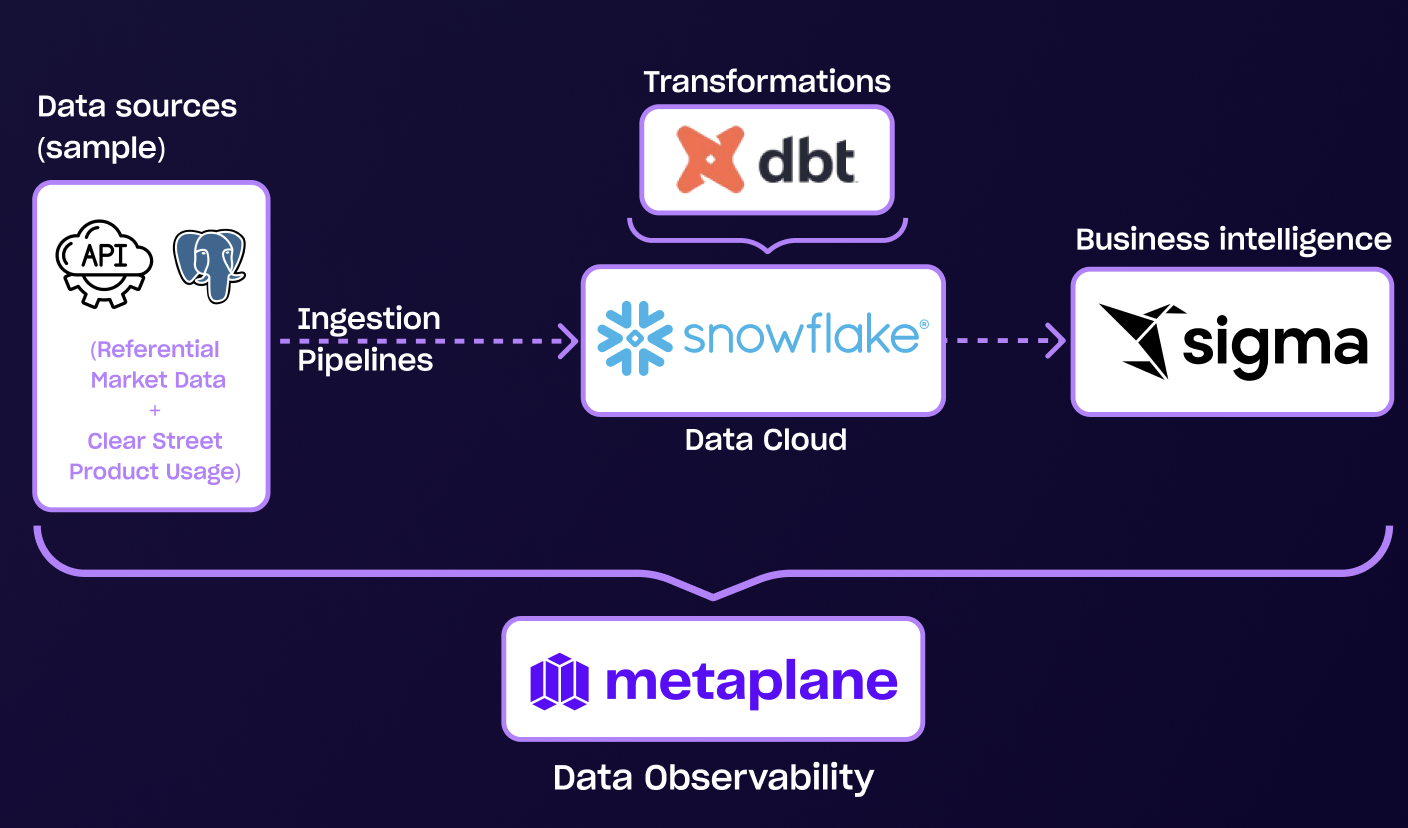
Introducing Metaplane
Prior to evaluating Metaplane, David and his team had been proactively working on data quality checks to feel confident that they were providing the best possible product for internal stakeholders, including row count checks between their transactional source database and target warehouse, Snowflake.
❝Data quality is something that people don’t think about as much (as other pieces of a data stack). Of course it has to be correct, but how do you ensure it? Those are the types of conversations we had.”
Although there weren’t any pressing data quality issues, David knew that the possibility of issues being created as they scaled out their data stack and strategy would only increase, and using his close working relationship with Shawn, also knew that the benefit of finding data quality issues would extend beyond the DATA team.
In Clear Street’s prime brokerage platform, a listed price being off by even a penny leads to valuations being off by 100s of dollars, and when multiplied by hundreds of users and transactions, can result in significant trading errors. Customers are the number one priority; The FACT team manages the critical referential data used for decision making through Clear Street’s platform, so it made sense to include referential data considerations into their evaluation of a data observability solution.
❝With referential data, there are hundreds of attributes for each instrument. If you’re off by 1, it can change the value dramatically down the pipe, and that’s just on that instrument itself. You can look at referential data as your building blocks (for a brokerage platform) - if the building blocks aren’t stable, then the building won’t be stable, and everything will topple over.”
In finding a data observability solution, Clear Street wanted something that not only included robust data quality monitors, but also had lineage built from integrations with their current and future data stack to better root cause issues and understand impact. With that in mind, the teams evaluated several solutions, including both open-source products and other paid tools, before deciding on Metaplane.
❝We want to be (data) rockstars, which means that we want our data to be good. Having ‘good data’ means resolving data issues before they become a hard-to-fix problem that requires tedious manual investigations.”
Evaluating Data Observability
In the process of choosing Metaplane as their Data Observability solution to make sure that this would be a worthwhile purchase for Clear Street, the team went through a rigorous evaluation. From the beginning, they were able to integrate existing pieces of their data stack, including Snowflake and Sigma, and set up data quality monitors, citing the “an easily navigable UI” in addition to existing integrations, as a reason for the accelerated implementation.
Using David and Shawn’s deep expertise of the data architecture that they had established, they were able to target critical objects with Metaplane’s data quality monitors, tracking data quality metrics ranging from freshness, row count, cardinality, and numeric distributions to string formatting for key fields including ticker symbols. The team now has over 300 monitors, which allowed them to capture incidents that represented actual customer issues:
❝We had a client that began creating an extraordinarily high number of records (compared to the typical usage of the Clear Street platform), and we were able to notice this with the Metaplane row count monitor. This allowed us to proactively reach out to understand how they were using our platform, and uncovered a fire drill that they were dealing with.”
Column level lineage was another key factor in their decision to purchase Metaplane. From the teams’ experience, they knew that when a data issue was found, they’d need to trace upstream through queries and ETL scripts to understand the root cause. Beyond that, their expanded use of Sigma Workbooks also meant that they had a desire to understand whether an issue was affecting multiple business intelligence reports. As they continued to use Metaplane and evolve their data stack, they uncovered an additional use case for lineage:
❝We had a project to deprecate a legacy database that was still in use. It’s been hard to track who’s using it where and we want to shut it down gracefully without impacting anyone still using it, and it’s been helpful to have a dependency graph to help with our deprecation analyses.”
As you can already tell, Clear Street is very forward-thinking when it comes to their data strategy, and wanted a Data Observability tool to keep pace with their growth, both in terms of integrations as well as innovative features.
- dbt: They’ve begun to expand the number of transformation models, and have Metaplane’s dbt job duration monitors on top of their projects, as a layer of security to make sure that a job failure, and not modeling mishap, can be immediately ruled out in the case of a data quality issue.
- Fivetran: The team at Clear Street is currently evaluating Fivetran among other data pipeline vendors to distribute some of the ingestion workload, and has already integrated Fivetran into Metaplane to monitor connector performance to instill confidence in a high integrity data replication solution.
In addition to being ready to integrate with popular tools from the modern data stack, David, Shawn, and their respective teams also had this to say about interactions with the team:
❝There’s always room for growth, and the growth that we’ve seen from Metaplane’s product to date has been very reassuring.”
Clear Street Successes
Several months from beginning their evaluation of Metaplane and other data observability solutions, the team at Clear Street has seen:
- The deployment of over 300+ data quality monitors
- Captured a critical incident that sparked a proactive customer success conversation about platform usage
❝It’s been very interesting to see how the models have established (data quality) thresholds (from our data) and watch how they’ve updated over time (as our data changes).”
Moving forward, they’ll continue to grow their data strategy to leverage more dbt when possible, implement an ingestion tool for data sources that it makes sense for, and continue instilling best practices in the team. If being at a company with a stellar data team and technology-first approach sounds like something you’d be interested in, you can find a list of open roles here.