What is data accuracy? Definition, examples, and best practices
Data accuracy isn't something for just your data team to care about—it has implications across your entire organization. Read on to learn what data accuracy is, common examples, and best practices for keeping your data accurate throughout your pipeline.

If you care about whether your business succeeds or fails, you should care about data accuracy. Data accuracy is important because it has an impact on your company's bottom line. Unfortunately, that impact often goes undetected—until it’s too late.
Say your business uses data for operational purposes, and your data is inaccurate. You could upset an entire segment of customers whose names you got wrong in an email—damaging your reputation and losing their trust. Or, you could lose profitable sales because you inadvertently listed an in-demand item as “out of stock” on your ecommerce website.
If your business uses data for decision-making purposes, on the other hand, and your data is inaccurate, it could have profound consequences. As an example, imagine using inaccurate market data to make a business decision about where to open your next location, only to find out that the region you chose has a median income too low to afford your products or services.
Now that you know why data accuracy matters, let’s dive into exactly what it means. In this blog post, you’ll find a definition, three examples of inaccurate data, and four methods for measuring data accuracy.
What is data accuracy?
Data accuracy is one of ten dimensions of data quality, and one of three dimensions that influence data integrity. Data is considered accurate if it correctly describes the real world. Ask yourself: Do the entities actually exist in your data collection? Do they have the attributes you describe in your data model? Do events occur at the times and with the attributes you claim? Accuracy needs to be examined at each level of abstraction in your data system.
Ensuring data consistency is crucial for maintaining data accuracy. Inconsistencies can indicate inaccuracies within datasets, leading to discrepancies in analyses, such as customer location distribution. Establishing standardized formats helps maintain data consistency across all datasets.
Unlike other data quality dimensions like timeliness or consistency, accuracy requires comparing your data to external reality. That makes it one of the trickiest dimensions to measure programmatically, but also one of the most crucial.
Data accuracy vs. data integrity
Data accuracy and data integrity are two closely related concepts in the realm of data management, but they serve different purposes. Data accuracy refers to the correctness and precision of data, ensuring that it reflects the real-world events or objects it is intended to describe. For instance, if a customer’s address is recorded accurately, it means the address in the database matches the actual physical address.
On the other hand, data integrity focuses on maintaining the consistency, trustworthiness, and reliability of data throughout its lifecycle. This involves ensuring that data remains unaltered and intact from its creation to its archival. Data integrity encompasses various aspects such as data validation, error detection, and prevention of unauthorized data modifications.
In essence, while data accuracy is concerned with the quality of the data itself, data integrity is about the processes and mechanisms that ensure the data remains accurate and reliable over time. Ensuring data accuracy is crucial for making informed decisions, reducing risks, and optimizing operations. Similarly, maintaining data integrity is essential for preventing data corruption, unauthorized modifications, and ensuring compliance with regulatory requirements.
Factors that affect data accuracy
Several factors can impact data accuracy, leading to inaccurate data that can compromise decision-making and operational efficiency. Here are some key factors:
- Human error: Typographical mistakes, misunderstandings, or omitted fields during manual data entry can lead to inaccuracies.
- System errors: Glitches, bugs, or outdated software can result in incorrect outputs or data corruption.
- Data transfer and migration: Errors can occur during data transfer or migration, leading to data truncation, discrepancies in formats, or complete data loss.
- Sampling errors: Flawed sampling methods or inadequate sample sizes can lead to outcomes that do not accurately represent the entire population.
- Outdated information: Changes in addresses, job changes, or company evolution can render data outdated and inaccurate.
- Incomplete data: Missing fields or unresponded survey questions can result in incomplete data.
- External interference: Hacking, unauthorized data alterations, or malware attacks can corrupt data.
- Bias and subjectivity: Personal beliefs, selective observation, or question phrasing can influence data.
- Measurement errors: Poorly calibrated tools or instruments can result in skewed data.
- Data source reliability: Unverified or questionable sources can provide inaccurate data.
- Integration of multiple data sources: Different methodologies or standards can result in inconsistencies.
- Environmental factors: Excessive heat, leading to data loss or corruption, can impact equipment and data accuracy.
Understanding these factors is crucial for implementing strategies to mitigate their impact and ensure high data accuracy.
Examples of inaccurate data
Inaccurate data comes in many forms across various industries. Let's look at some common examples:
Example 1: Missing or incorrect values
Imagine you’re a lead analytics engineer at Rainforest, an eCommerce company that sells hydroponic aquariums to high-end restaurants. Your data would be considered “bad data” / ”inaccurate data” if the number of aquariums shipped from the warehouse did not match the actual number sold as reported by your sales team, due to accidental manual data entry in the data source. The same would be true if the geographies assigned to each sales rep were not correct, or the dollar amount of a specific sale was off by a significant amount. These are but two examples of data inaccuracy.

Note: as more companies move toward automation in their “big data” strategy, poor data quality can cause negative downstream impacts for all uses of data from artificial intelligence to data analytics.
Example 2: Attribute errors
Your data may have the right entities, but wrong attributes. For instance, if customer addresses in your database have incorrect zip codes or if product descriptions list the wrong specifications, these are accuracy issues that can affect everything from shipping to customer satisfaction.
Example 3: Outdated information
Data that was once accurate but has become outdated is another form of inaccuracy. This could be pricing information that hasn't been updated, inventory levels that don't reflect recent sales, or customer contact information that has changed.
Example 4: Duplicated records
When your system contains multiple records for the same real-world entity (like duplicate customer profiles), this creates inaccuracy through redundancy. These duplicates can lead to errors in aggregations, reporting, and operational decisions.
How do you measure data accuracy?
Measuring data accuracy requires a systematic approach that combines automated checks with human validation, focusing on the number of inaccuracies in relation to the total data points. Here are several methods that data teams commonly use:
1. Reference data comparison
Compare your data values against authoritative reference sources or "golden records." For example, validate customer addresses against postal service databases or product information against manufacturer specifications.
```sql
-- Validate US zip codes against reference table
SELECT
COUNT(*) as invalid_zip_count
FROM customer_addresses
WHERE zip_code NOT IN (SELECT zip_code FROM reference.valid_us_zipcodes);
```
2. Cross-system validation
Check for consistency between systems that should contain the same data. If your CRM and marketing automation platform have different email addresses for the same customer, at least one is inaccurate.
```sql
-- Compare email addresses between CRM and marketing systems
SELECT
crm.customer_id,
crm.email as crm_email,
marketing.email as marketing_email
FROM crm.customers crm
JOIN marketing.contacts marketing ON crm.customer_id = marketing.customer_id
WHERE crm.email != marketing.email;
```
3. Statistical analysis
Use statistical methods to identify outliers and anomalies that may indicate inaccurate data. For numerical data, techniques like z-scores can help flag values that deviate significantly from the norm.
```sql
-- Identify potential pricing errors using z-score
WITH price_stats AS (
SELECT
AVG(price) as avg_price,
STDDEV(price) as stddev_price
FROM products
WHERE category = 'electronics'
)
SELECT
product_id,
product_name,
price,
(price - avg_price) / NULLIF(stddev_price, 0) as z_score
FROM products, price_stats
WHERE category = 'electronics'
AND ABS((price - avg_price) / NULLIF(stddev_price, 0)) > 3;
```
4. Business rule validation
Apply domain-specific business rules to identify logically impossible or implausible values. For example, a rule might flag orders with ship dates earlier than order dates.
```sql
-- Find orders with impossible date relationships
SELECT
order_id,
order_date,
ship_date
FROM orders
WHERE ship_date < order_date
OR (delivery_date IS NOT NULL AND delivery_date < ship_date);
```
How to ensure data accuracy
Ensuring data accuracy requires a wholistic approach combining preventative measures with detection and correction capabilities.
1. Implement data validation at collection points
The best way to maintain accuracy is to prevent inaccurate data from entering your systems in the first place. This means implementing validation rules at data entry points:
- Add form validation for user inputs (e.g., email format validation, required fields)
- Implement dropdown menus instead of free text fields where possible
- Use data type constraints and range checks on fields
2. Establish data governance processes
Create clear policies around data management:
- Define data ownership and stewardship responsibilities
- Establish standard operating procedures for data handling
- Document expectations for data quality and accuracy
- Create formal processes for managing data changes and updates
3. Use anomaly detection for monitoring
One powerfully scalable approach to ensuring data accuracy is through anomaly detection (sometimes called outlier analysis), which helps you identify unexpected values or events in a dataset.

Using the example of a sale that was reported inaccurately, anomaly detection software would notify you instantly if that value was outside of the normal range. The software recognizes outliers because its machine learning model learns from your historical metadata.
Here's how anomaly detection helps Andrew Mackenzie, Business Intelligence Architect at Appcues, perform his role:
"The important thing is that when things break, I know immediately—and I can usually fix them before any of my stakeholders find out."
In other words, you can say goodbye to the dreaded "WTF" messages from your stakeholders. Automated, real-time anomaly detection acts like a vigilant colleague who's always watching your data.
4. Implement regular data cleansing
Even with preventative measures, inaccurate data will find its way into your systems. Establish regular data cleansing routines:
- Deduplicate records on a scheduled basis
- Standardize and normalize values (like address formats)
- Enrich data with third-party sources to improve accuracy
- Remove or archive outdated information
Data cleansing is crucial for maintaining high-quality data, ensuring that your data is accurate, consistent, complete, and timely for reliable analysis and effective decision-making.
5. Train your team
Human error is a leading cause of data inaccuracy. Invest in training:
- Educate data entry personnel on the importance of accuracy
- Train analysts on how to identify potentially inaccurate data
- Create a culture that values data quality
Data collection and integration
Data collection and integration are critical components of ensuring data accuracy. Data collection involves gathering data from various sources, while data integration involves combining data from multiple sources into a unified view. To ensure data accuracy during data collection and integration, consider the following best practices:
- Use data validation tools: Automated software tools can validate information upon data ingestion, ensuring that only accurate data enters your systems.
- Establish a data governance team: A dedicated governance team can define and enforce data quality standards, ensuring consistent and accurate data management practices.
- Conduct regular data audits: Periodic reviews can help maintain high data integrity and identify inaccuracies, allowing for timely corrections.
- Train staff: Adequate training is essential for people involved in data processing to understand how to maintain accurate data and recognize potential inaccuracies.
- Use data profiling tools: Tools can help review and analyze existing data to identify inconsistencies, anomalies, and irregularities, ensuring that data remains accurate and reliable.
By implementing these practices, organizations can significantly improve their data quality and ensure that their data remains accurate and useful for decision-making.
Common data accuracy challenges
Despite the importance of data accuracy, several challenges can arise, including:
- Inaccurate data entry: Human or schematic errors can result in inaccurate content or form, leading to poor data quality.
- Data integration and transformation challenges: Inaccuracies can occur during data transformation or alignment and reconciliation difficulties, especially when integrating data from multiple sources.
- Data governance and management hurdles: Poor data governance practices can jeopardize the accuracy of entire datasets, leading to data quality issues.
- Technological limitations: System glitches, data storage and retrieval issues, and insufficient data quality tools and resources can contribute to data inaccuracies.
To overcome these challenges, organizations can adopt various strategies, such as leveraging data validation tools, implementing quality assurance methods, and engaging stakeholders in data management. By addressing these challenges proactively, organizations can ensure high data accuracy and maintain the integrity of their data assets.
Creating a data accuracy strategy
To systematically address data accuracy, consider implementing these strategic elements:
- Data profiling: Regularly analyze data to understand its structure, relationships, and quality issues
- Accuracy SLAs: Establish service level agreements for data accuracy in critical systems
- Root cause analysis: When accuracy issues occur, investigate the underlying causes rather than just fixing symptoms
- Continuous improvement: Use insights from accuracy issues to improve data processes and systems
- Stakeholder feedback loops: Create channels for business users to report suspected accuracy issues
Final thoughts
Data accuracy is fundamental to building trust in your data and making sound business decisions. By implementing robust validation, monitoring, and correction processes, you can significantly improve the accuracy of your organization's data assets.
To take anomaly detection for a spin and put an end to poor data quality, sign up for Metaplane's free-forever plan or test our most advanced features with a 14-day free trial. Implementation takes under 30 minutes.
Editor's note: this article was originally published in May 2023, and updated for accuracy and freshness in March 2025.
Table of contents
Tags
...

...

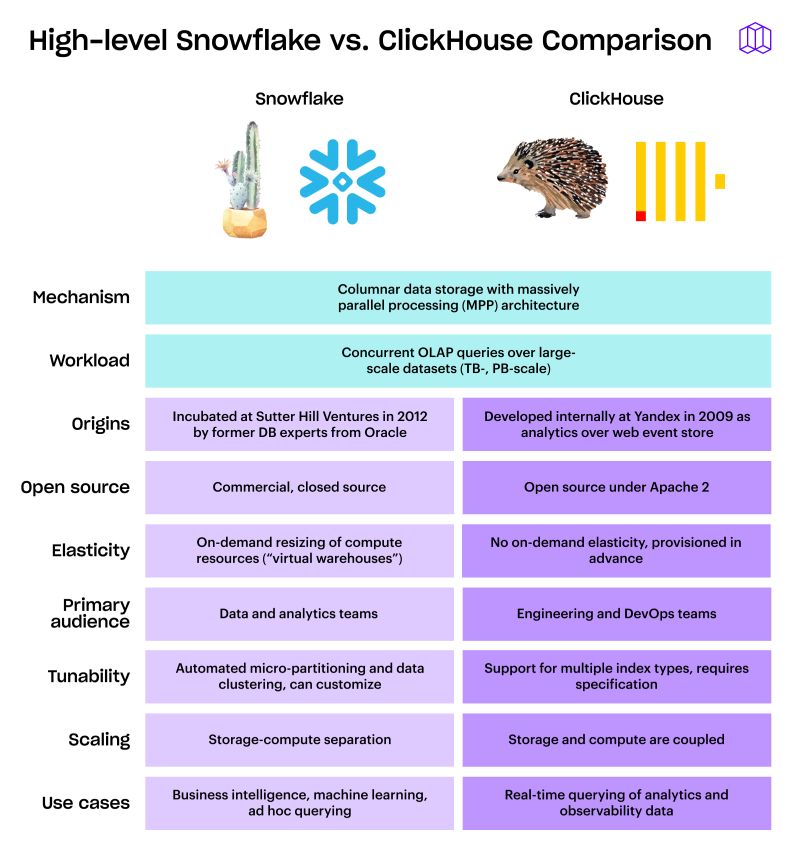


