What is data consistency? Definition, examples, and best practices
Data consistency is one of the ten dimensions of data quality. In this post, you'll learn what it means, why it matters, and how it's measured.
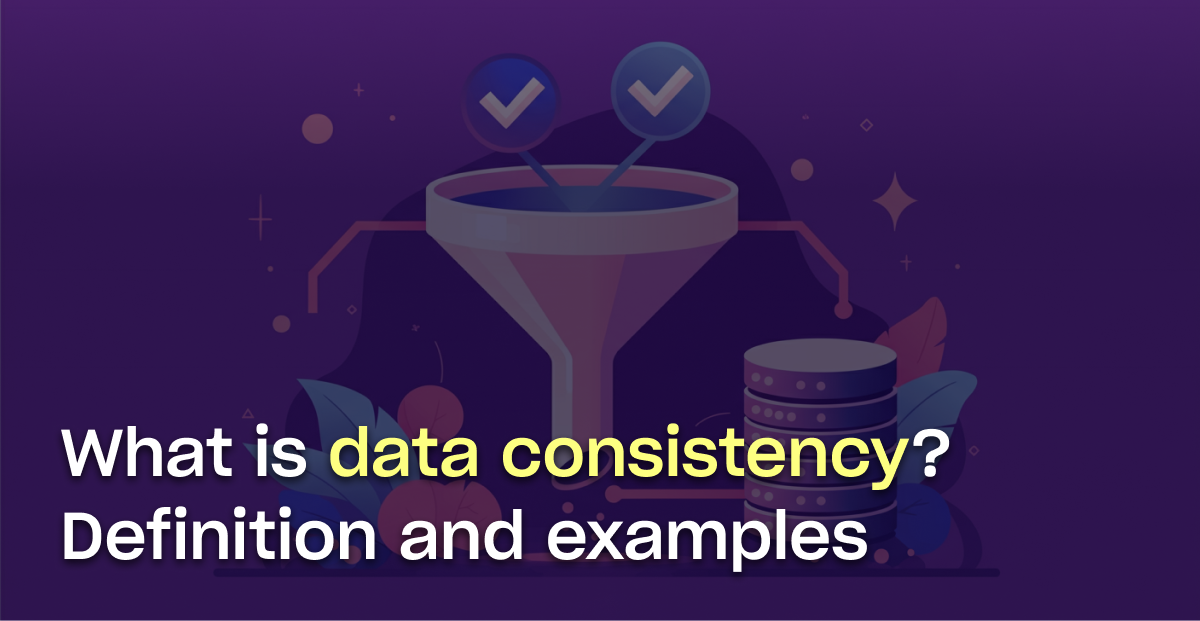
If you care about whether your business succeeds or fails, you should care about data consistency. Consistent data is important because it has a huge impact on your bottom line. Unfortunately, that impact often goes undetected—until it’s too late.
Say your business uses data for operational purposes, and your data is inconsistent, leading to low data integrity. You could inadvertently send an automated renewal email to a customer who’s at risk of churning because your “account status” wasn’t identical across Gainsight and Salesforce, leading to lack of concurrency.
If your business uses data for decision-making purposes, on the other hand, and your data is inconsistent, it could cost you. As an example, imagine deciding to double-down on digital advertising because your return on ad spend appeared high, when according to a second source you’re barely breaking even.
Now that you know why data consistency matters, let’s dive into exactly what it means. In this blog post, you’ll find a definition, examples, and four methods for measuring data consistency.
What is data consistency?
Data consistency ensures accuracy and coherence across various systems, which is crucial for reliable decision-making and data integrity. It's one of the ten dimensions of data quality.
Data is considered consistent if two or more values in different locations are identical. Ask yourself: Is the data internally consistent? If there are redundant data values, do they have the same value? Or, if values are aggregations of each other, are the values consistent with each other?
Conversely, data inconsistency can undermine these benefits, leading to unreliable decision-making and compromised data integrity.
What causes inconsistent data?
Data inconsistency can arise from various sources, including human error, system glitches, and integration issues. When your dashboards suddenly show conflicting information, these are likely the culprits behind your data headaches.
- Human error: Manual data entry mistakes happen to the best of us. That customer name entered differently across systems? It's creating join failures that ripple through your entire data pipeline.
- System glitches: Technical issues don't announce themselves. A system crash during updates leaves your data in limbo – partially processed and wholly unreliable for those critical morning reports.
- Integration issues: When your data comes from multiple sources, synchronization becomes critical. One system updates while another doesn't, and suddenly you're comparing yesterday's numbers with today's data.
- Lack of data governance: Without clear rules of the road, different departments create their own standards. Marketing uses one naming convention, Sales another – and your analysis treats them as entirely different entities.
- Inadequate data validation: Your systems should be gatekeepers, not open doors. Without proper validation checks, duplicate records multiply, impossible values sneak in, and you're left explaining why the numbers "just don't add up."
Types of data consistency
Data consistency isn't one-size-fits-all. Depending on your use case, you might need ironclad guarantees or be able to tolerate some wiggle room. Understanding these different models helps you make the right tradeoffs between accuracy, performance, and user experience. After all, the consistency level that works for a banking transaction won't be the same as what you need for a product recommendation engine.
There are several types of data consistency, each with its own characteristics and use cases:
- Strong consistency: Ensures that all copies of data are identical and up-to-date at all times. This type of consistency is crucial for applications where real-time accuracy is essential, such as financial transactions. When your payment processing system needs to know exactly how much money is in an account, this is your go-to.
- Eventual consistency: Allows for temporary inconsistencies but ensures that data will eventually become consistent. This approach is often used in distributed systems where immediate consistency is not critical, but eventual accuracy is required. Think social media likes or e-commerce product reviews—a slight delay is acceptable.
- Weak consistency: Does not guarantee consistency but may provide some level of consistency. This type of consistency is suitable for applications where occasional discrepancies are acceptable, and performance is prioritized over accuracy. Analytics dashboards showing approximate counts often fall into this category.
- Causal consistency: Ensures that the order of events is preserved, even if data is not immediately consistent. This type of consistency is important for applications where the sequence of operations matters, such as collaborative editing tools. It prevents that frustrating scenario where changes seem to appear out of order.
- Sequential consistency: Ensures that all operations are executed in a consistent order. This type of consistency is essential for applications where the order of operations must be maintained to ensure correct results, such as in database transactions. Your database writes need to happen in the exact order you intended.
Real-world examples of inconsistent data
Imagine you’re a lead analytics engineer at Rainforest, an ecommerce company that sells hydroponic aquariums to high-end restaurants. An example of data inconsistency here would be if the engineering team records aquarium models from database transactions that don’t match the models recorded by the sales team from the CRM.

Another example would be if the monthly profit number is not consistent with the monthly revenue and cost numbers. Some of the ways that this could happen would be if you have concurrent workloads, which could be in the form replication pipelines themselves, or downstream SQL transformations that lead to additional nodes (forks) in your end to end pipelines. The solution to all of this would be proper data management, starting with measuring for data consistency.
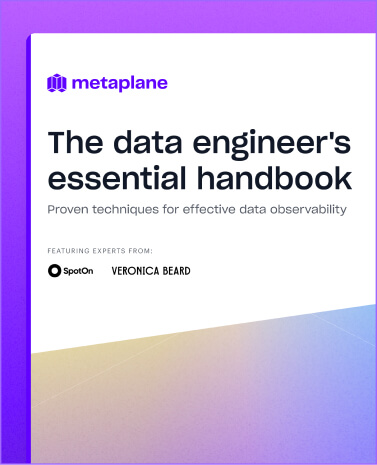
How do you measure data consistency?
To test your any data quality dimension, you must measure, track, and assess a relevant data quality metric. In the case of data consistency, you can measure the number of passed checks to track the uniqueness of values, uniqueness of entities, corroboration within the system, or whether referential integrity is maintained. Codd’s Referential Integrity constraint is one example of a consistency check.
Practical consistency checks to implement today
Here are some examples of consistency checks you can implement today, and example scenarios of when you might use them.
1. Column-to-column comparisons
This check identifies records where the same attribute exists in multiple tables but has different values. It's particularly useful for catching sync issues when data is duplicated across systems or when denormalization has gone wrong. In this example, we're finding users whose email addresses don't match between the users and profiles tables, which could cause authentication problems or missed communications.
-- Check if user.email matches profile.email
SELECT COUNT(*)
FROM users u
JOIN profiles p ON u.id = p.user_id
WHERE u.email != p.email;
2. System-to-system validations
This check compares aggregate metrics between different systems to ensure they're aligned. It's crucial for financial reconciliation and cross-system integrity. In this example, we're comparing the total opportunity amount in Salesforce with the total deal revenue in our data warehouse. Even small differences can indicate missing records, transformation errors, or sync timing issues that could affect revenue reporting.
-- Compare Salesforce opportunities to data warehouse
WITH sf_data AS (
SELECT SUM(amount) AS total_amount FROM salesforce.opportunities
),
warehouse_data AS (
SELECT SUM(revenue) AS total_amount FROM warehouse.deals
)
SELECT
sf_data.total_amount AS salesforce_amount,
warehouse_data.total_amount AS warehouse_amount,
ABS(sf_data.total_amount - warehouse_data.total_amount) AS difference
FROM sf_data, warehouse_data;
3. Aggregation validation
This check verifies that pre-computed aggregates match what you'd get by summing the detailed records. It's essential for catching ETL errors, partial data loads, and aggregation logic bugs. The query uses a small tolerance (0.01) to account for potential floating-point precision issues, while still identifying meaningful discrepancies between monthly sales totals and their constituent daily sales.
-- Verify monthly_sales matches daily_sales
SELECT
m.month,
m.total_sales AS monthly_total,
SUM(d.sales) AS sum_of_daily,
ABS(m.total_sales - SUM(d.sales)) AS difference
FROM monthly_sales m
JOIN daily_sales d ON DATE_TRUNC('month', d.date) = m.month
GROUP BY m.month, m.total_sales
HAVING ABS(m.total_sales - SUM(d.sales)) > 0.01;
4.Change-over-time consistency
Flag logical inconsistencies in time seriesThis check identifies temporal inconsistencies where values violate logical constraints. For metrics that should only increase over time (like cumulative counters), any decrease indicates a data problem. The query uses the SQL window function LAG() to compare each day's cumulative user count with the previous day, flagging records where the count has impossibly decreased, which could indicate data loss, incorrect backfills, or counting logic errors.
-- Detect impossible decreases in cumulative metrics
SELECT
date,
cumulative_users,
LAG(cumulative_users) OVER (ORDER BY date) AS previous_day,
cumulative_users - LAG(cumulative_users) OVER (ORDER BY date) AS difference
FROM daily_metrics
WHERE cumulative_users < LAG(cumulative_users) OVER (ORDER BY date);
Ensuring data consistency with Metaplane
One way to ensure data consistency is through anomaly detection, sometimes called outlier analysis, which helps you to identify unexpected values or events in a data set.
Using the example of two numbers that are inconsistent with one another, anomaly detection software would notify you instantly when data you expect to match doesn’t. The software knows it’s unusual because its machine learning model learns from your historical metadata.
Here’s how anomaly detection helps Andrew Mackenzie, Business Intelligence Architect at Appcues, perform his role:
“The important thing is that when things break, I know immediately—and I can usually fix them before any of my stakeholders find out.”
In other words, you can say goodbye to the dreaded WTF message from your stakeholders. In that way, automated, real-time anomaly detection is like a friend who has always got your back.
Data consistency FAQ
How does data consistency differ from application consistency vs strong consistency?
For purposes of this article, we've be focused solely on data consistency as it relates to the actual values themselves. You may see some overlap with Strong Consistency and Application Consistency, other terms in the data space:
- Strong Consistency: You may run into this term when looking up database consistency as well, particularly in complex database system architectures. Strong consistency is all about ensuring that everyone in a distributed system is on the same page when it comes to data, and includes the concepts from CAP theorem. It means that no matter which node or replica you're looking at, they all have the most up-to-date view of the data at any given time. It's like making sure everyone sees things happening in the same order, as if there's only one copy of the data. So, when you read something, you can always trust that you're getting the latest version. Achieving strong consistency usually involves using coordination mechanisms like distributed transactions or consensus algorithms to make sure the data stays intact and in sync across the entire distributed system. Note: It’s important here to maintain atomicity of timestamps to ensure you don’t miss any data changes.
- Application Consistency: Application consistency refers to making sure that the data within an application (app), typically hosted in your database system, is in good shape and follows the rules and requirements set by that application. It's like ensuring that everything is in order and makes sense according to how the app is supposed to work. When an app is consistent, you can trust that the data is accurate, complete, and meets the specific rules or relationships defined by the app. It's all about making sure things run smoothly and produce reliable results. To achieve application consistency, developers need to implement checks and safeguards to validate data, handle errors effectively, and enforce the application's unique rules. Note: a crossover here in data governance may be to utilize data validation during a user’s data entry (e.g. email) to ensure that downstream usage of that field can be maintained.
For simplicity’s sake, when we’re referring to data consistency here, the concepts will be largely applicable to your data warehouse of choice.
To take anomaly detection for a spin and put an end to poor data quality, sign up for Metaplane’s free-forever plan or test our most advanced features with a 14-day free trial. Implementation takes under 30 minutes.
Editor's note: This article was originally published in May 2023 and updated for accuracy and freshness in March 2025.
Table of contents
- What is data consistency?
- What causes inconsistent data?
- Types of data consistency
- Real-world examples of inconsistent data
- Data consistency FAQ
Tags
...

...

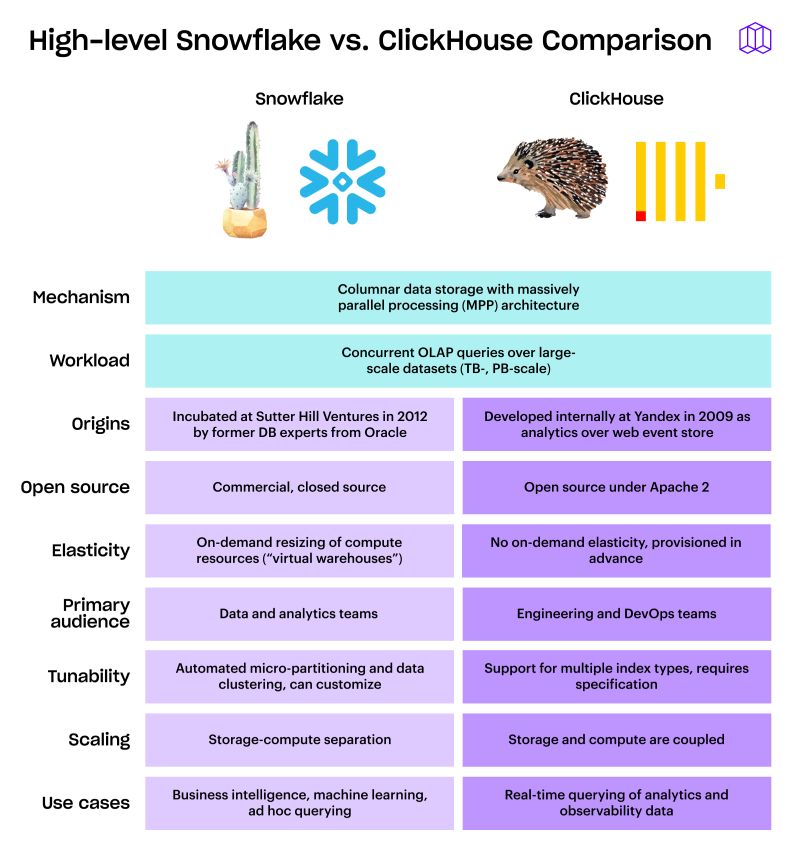


