Data Mesh vs. Data Lake: Key Differences and Which to Choose in 2023
As more and more organizations rely on data to power their growth, the question of how to store, process, and manage that data has become increasingly pressing. Two popular options are Data Mesh and Data Lake architectures. Both approaches have their advantages and drawbacks, and selecting the right one for your organization should be a thoroughly considered choice.
In this blog post, we'll explore the key differences between these architectures and help you understand which one might be right for your organization.
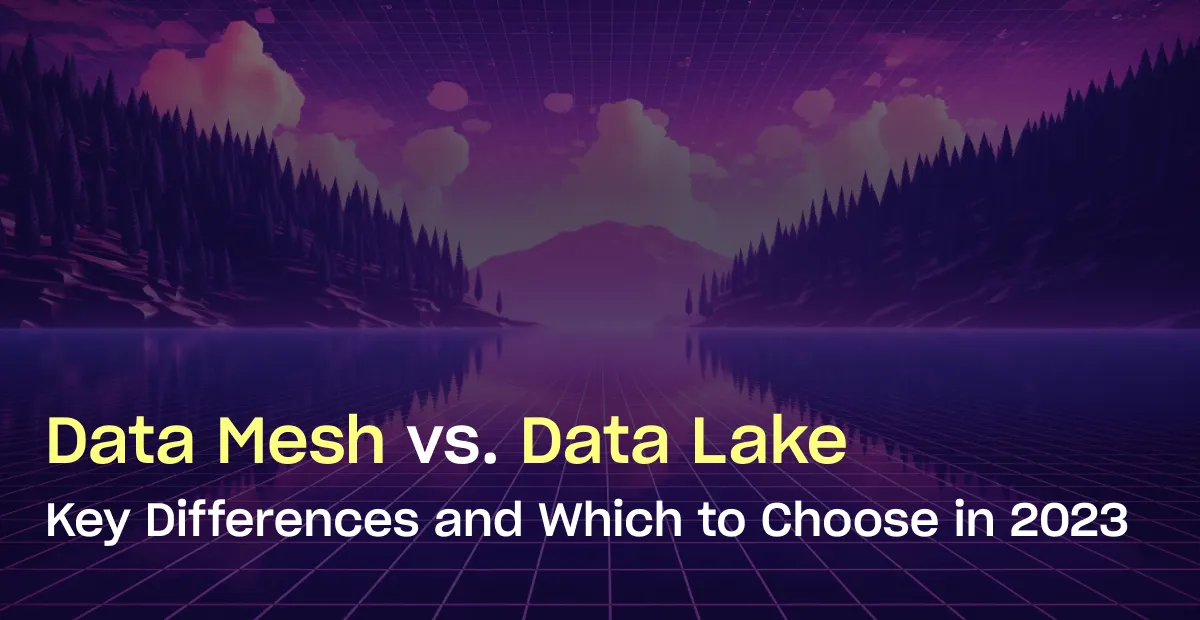
Before diving into the details of Data Mesh and Data Lake architectures, let's briefly define these terms.
A Data Mesh is a novel data platform design paradigm that emphasizes domain-driven decentralized data management, self-serve data infrastructure, and a federated governance model.
A Data Lake is a centralized repository for storing raw data that can be processed and analyzed for different business purposes. Both architectures aim to provide a scalable, flexible and adaptable data platform that meets the needs of an organization.
Choosing the right architecture for your data platform is increasingly important as data products become central to business strategy.
Understanding Data Mesh
Effective data management requires a flexible and adaptable platform that can meet the specific needs of your organization. Data Mesh is a novel paradigm that has emerged to address the limitations of traditional data platforms.
At a high level, Data Mesh architecture emphasizes a domain-driven approach to data management. This means that data is owned and managed by each domain, such as finance, marketing, or logistics. Each domain operates its own infrastructure to create, store, and manipulate data, which can then be integrated into a federated structure.
One advantage of Data Mesh is increased scalability, particularly when dealing with complex data models. Traditional data platforms typically struggle to handle complex data models, but Data Mesh's decentralized approach allows for better granularity and ownership of data. This can result in faster insights and data-driven decision-making.
However, Data Mesh architecture also presents some challenges, such as increased complexity in data modeling and high infrastructural costs. Ensuring high-quality data is also a challenge because ownership is distributed across domains, making it more difficult to enforce standardization and data governance policies.
This is where data observability becomes essential. Metaplane's tools, such as automated data quality checks and change notifications, can help you ensure that your data is high quality and that changes are visible across the entire architecture.
Overall, Data Mesh architecture is a good choice for organizations with complex data models and a need for increased scalability. However, careful planning, high-quality infrastructure, and stringent governance policies are essential to ensure it works effectively.
Understanding Data Lake
While Data Mesh architecture emphasizes a decentralized approach to data management, Data Lake architecture takes a centralized approach. In a Data Lake architecture, all raw data is stored in a centralized repository. From there, it can be processed and analyzed for different business purposes.
Data Lake architecture has many benefits, including cost-effective storage of large quantities of data, flexible storage of multiple data types, and scalable analytics capabilities. However, if not managed correctly, data silos can form, making it difficult to integrate data across domains. Strong data cleansing and transformation practices are also essential to ensure that data is of high enough quality to be useful.
Data observability is also essential in a Data Lake architecture. This approach makes it easier to identify data silos and ensure that data is being transformed and cleaned properly. With Metaplane's tools, you can monitor the quality and consistency of your data across the entire architecture.
Data Lake architecture is well suited for organizations that need to store and analyze large quantities of different data types. However, governance practices and cleansing procedures must be in place to ensure that data is useful for business purposes.
Data Mesh vs Data Lake: Key Differences
The primary difference between Data Mesh and Data Lake architecture is in their design principles. Data Mesh emphasizes a decentralized approach to data management, while Data Lake takes a centralized approach. This difference results in different approaches to data governance and management.
In Data Mesh, data is organized into domains with each domain responsible for managing its own data infrastructure. This approach allows for better ownership and governance of data, but it also presents challenges in ensuring standardization and quality across domains.
In Data Lake architecture, all data is stored in a centralized repository, which can make it easier to integrate data. However, this approach requires more governance and cleansing to ensure that the data is of high quality.
Data Mesh | Data Lake | |
---|---|---|
Design Principle | Decentralized approach with data divided among different business domains. | Centralized approach where all data is stored in a central repository. |
Data Management | Each domain owns and manages its own data, potentially increasing scalability and granularity of data. | Raw data from various sources is stored centrally, allowing for large-scale storage and processing. |
Data Governance | Data governance is distributed, with each domain taking responsibility for its own data quality and standardization. | Central governance and cleansing practices are needed to ensure data quality. |
Benefits | Better granularity and ownership of data, potentially leading to faster insights. | Cost-effective storage of large quantities of data, flexibility in storing multiple data types, and scalable analytics capabilities. |
Challenges | Increased complexity in data modeling and potential increase in infrastructure costs. Standardization and data quality can be challenging due to distributed ownership. | Potential for data silos making integration across domains difficult. Strong data cleansing and transformation practices are required to ensure high-quality data. |
Factors to Consider When Choosing Between Data Mesh and Data Lake
When deciding between Data Mesh and Data Lake architecture, several factors must be considered. The size and complexity of the organization's data models are essential factors to consider. Data Mesh is well suited for organizations with complex data models, but it requires careful planning, infrastructure, and governance policies to be effective.
Additionally, existing data infrastructure and transformation needs must also be taken into account. Data Lake architecture is well suited for organizations that need to store and analyze large quantities of different data types. However, governance practices and cleansing procedures must be in place to ensure that data is useful for business purposes.
Finally, data observability is a critical factor to consider when deciding between Data Mesh and Data Lake architecture. With Metaplane's monitoring and troubleshooting tools, you can ensure that your data is of high quality and consistency across the entire architecture, regardless of which approach you choose.
Case Studies: Data Mesh and Data Lake in Action
To help illustrate the application of Data Mesh and Data Lake architectures, let's explore some real-world examples:
Data Lake Case Studies
Netflix, the streaming giant, extensively utilizes Data Lake architecture to manage its vast trove of data. With a large variety of data types ranging from viewer preferences, streaming logs, to customer service interactions, Netflix relies on their Data Lake to store and process this data efficiently. The centralized repository enables Netflix to gain granular insights and drive data-driven decision-making, enhancing customer experience and content strategy.
JPMorgan Chase, one of the largest banking institutions worldwide, implements a Data Lake architecture to handle its massive volume of data. This data includes transaction details, customer profiles, and market data. The centralization of this data in a Data Lake enables JPMorgan Chase to streamline its data processing and analytics, improving risk management, personalizing customer services, and facilitating informed business decisions.
Data Mesh Case Studies
Zalando, a major online fashion platform in Europe, has pioneered the application of the Data Mesh concept. With its diverse and complex system comprised of numerous autonomous teams, each managing their domain, Zalando's shift toward a Data Mesh architecture has maintained agility while ensuring each team's full ownership of their data. This approach has led to improved data quality and expedited decision-making.
Intuit, the financial software company known for products like TurboTax and QuickBooks, has begun implementing a Data Mesh architecture to better manage their increasingly diverse and distributed data sources. By allowing different teams to own and manage their respective data domains, Intuit has been able to increase data quality, reduce bottlenecks, and foster more effective cross-functional collaborations.
Conclusion: Which to Choose – Data Mesh or Data Lake?
Choosing between Data Mesh and Data Lake architecture is an essential decision for data leaders. Both approaches have their pros and cons, and the choice must be based on the specific needs of the organization.
Factors such as the complexity of data models, existing data infrastructure, transformation needs, and data observability must be taken into consideration when deciding between the two approaches. Both architectures require careful planning and governance policies to ensure that data is of high quality and consistent across the entire architecture.
In some cases, Data Mesh may be a better fit for organizations with complex data models, while Data Lake architecture may be better for organizations that need to store and analyze large quantities of different data types. However, regardless of which architecture you choose, data observability is an essential component of a successful data platform.
Metaplane's data observability platform is optimized for the modern data platforms and designed to help data teams overcome the challenges in data quality. To learn more, get started in minutes for free, or book a demo.
Looking to the future, data platforms will continue to play an essential role in business strategy. As organizations grow and expand, they will need to adopt flexible, scalable, and adaptable data platforms that can meet their specific needs. At Metaplane, we're committed to providing monitoring and troubleshooting tools that help data leaders ensure that their data platforms are of high quality and consistency, regardless of which architecture they choose.
Frequently Asked Questions (FAQs)
Q: What is a Data Mesh architecture?
A: Data Mesh is a novel data platform design paradigm that emphasizes domain-driven decentralized data management, self-serve data infrastructure, and a federated governance model.
Q: What is a Data Lake architecture?
A: In a Data Lake architecture, all raw data is stored in a centralized repository. From there, it can be processed and analyzed for different business purposes.
Q: What are the main differences between Data Mesh and Data Lake architecture?
A: The primary difference is in their design principles. Data Mesh emphasizes a decentralized approach to data management, while Data Lake takes a centralized approach.
Q: What factors should be considered when choosing between Data Mesh and Data Lake architecture?
A: Factors such as the complexity of data models, existing data infrastructure, transformation needs, and data observability must be taken into consideration when deciding between the two approaches.
Q: Why is data observability important in both Data Mesh and Data Lake architecture?
A: Data observability is essential to ensuring that data is of high quality and consistency across the entire architecture, regardless of which approach you choose.
Q: How does the choice between Data Mesh and Data Lake architecture impact data governance?
A: Data governance in a Data Mesh architecture is distributed across different domains, with each domain responsible for its own data. In a Data Lake architecture, data governance is more centralized, as all data is stored in one place.
Table of contents
Tags
...

...

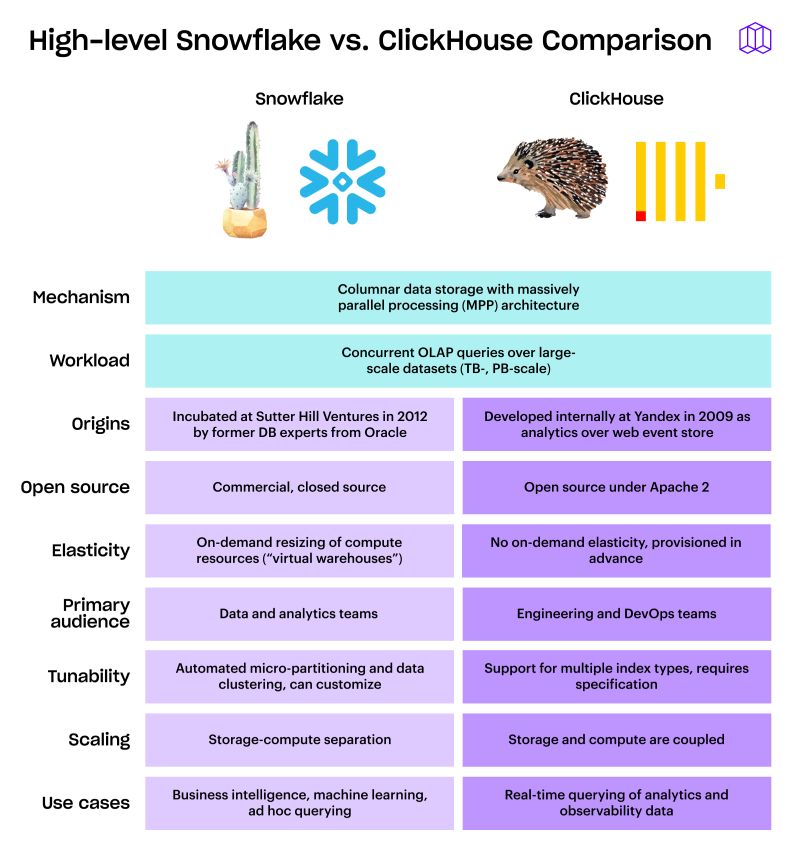


