What is Data Reliability? Definition, Examples, and Best Practices
Data reliability is crucial for making informed decisions, improving operational efficiency, and enabling data-driven initiatives. In this blog post, we'll explore what data reliability is, examples of unreliable data, how to measure data reliability, and best practices for ensuring data reliability.
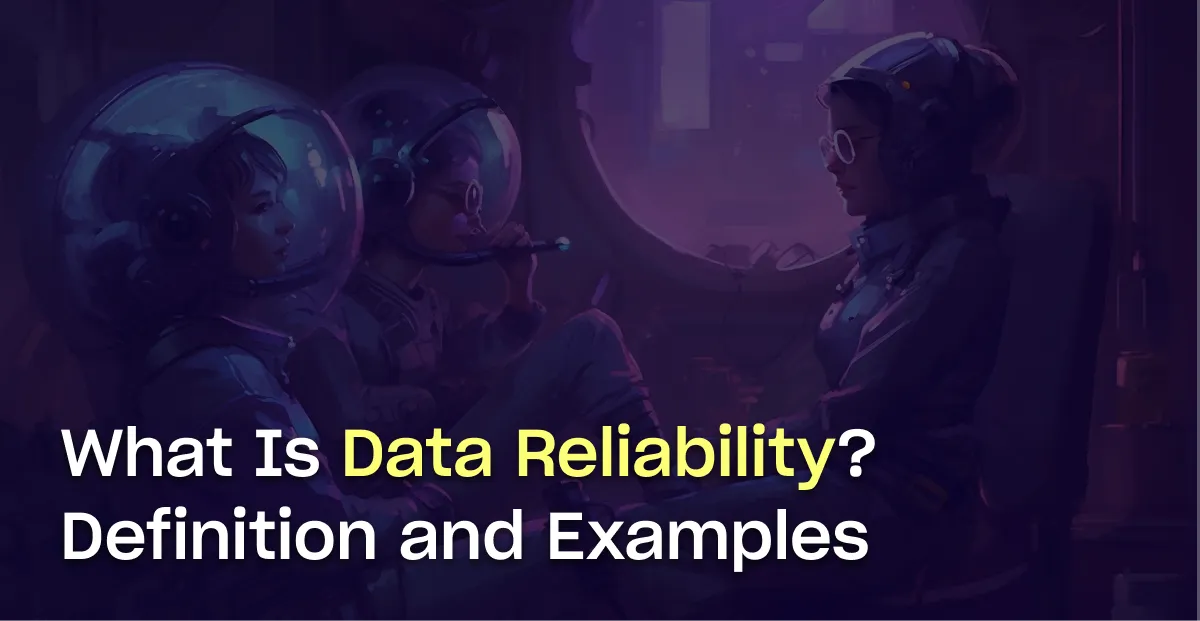
What is Data Reliability?
Data reliability is one of 10 dimensions of data quality, referring to the fitness of data for operational and decision-making purposes. Other examples of dimensions of data quality include usability, security, and validity. In other words, data reliability is the extent to which data is trusted by downstream stakeholders for their data use case. Each of these dimensions is important, but data reliability is the essential foundation for all data analytics and decision-making, particularily by business users.
Examples of Unreliable Data
Reliable data is essential for business analytics and decision-making, but unreliable data can lead to costly mistakes. While there are many ways data can become unreliable, here are three common examples:

- Incomplete data: When data is incomplete, it may be tempting to fill in the gaps with assumptions or approximations. However, this can lead to inaccurate results. For example, if you're analyzing monthly revenue data and some months are missing, you may be tempted to average the remaining months to fill the gap. But this can lead to incorrect revenue projections.
- Inconsistent data: When data is inconsistent, it can create confusion and mistrust. For example, if you're analyzing e-mail addresses and some addresses appear in different formats, it can be difficult to know which format is correct.
- Missing data: When data is missing, it can lead to incorrect conclusions or assumptions. For example, if you're analyzing churn data and some churn reasons are missing, you may assume that those customers simply lost interest when in fact there may have been other reasons why they left.
How Do You Measure Data Reliability?
To ensure data reliability, it's important to have real-world metrics that your team uses to measure data reliability over time. Here are three example metrics:
- Completeness rate: This metric measures the percentage of complete data in a given dataset. In our activated_users table, for example, a completeness rate of 100% would mean that every row has all necessary fields.
- Consistency rate: This metric measures the percentage of consistent data in a given dataset. In our daily_revenue table, for example, a consistency rate of 100% would mean that every revenue value is in the same format.
- Accuracy rate: This metric measures the percentage of accurate data in a given dataset. In our activated_users table, for example, an accuracy rate of 100% would mean that every e-mail address is correct.
How to Ensure Data Reliability
Ensuring data reliability requires a continuous effort to maintain data quality. Here are three best practices for maintaining data reliability:
- Establish data quality policies: Establish clear policies and guidelines for data entry, data validation, and data handling. Make sure that everyone on your team is aware of these policies and that they are followed consistently.
- Utilize anomaly detection: Anomaly detection can help identify changes or inconsistencies in your data that may indicate data quality issues. Incorporating anomaly detection via your data observability tool helps maintain data reliability and prevent costly mistakes.
- Choose accessible data tools: In some cases, data is seen as unreliable due to tool failures. For example, a business intelligence tool outage may be immediately apparent to a user who receives a 404 error upon logging in, but when the warehouse that actually stores data is down, a user that only interacts with the business intelligence tool for anlaytics may mistakenly assume that blank dashboards they see indicates unreliable data.
By understanding data reliability, measuring its impact, and implementing best practices to ensure it, you can maintain trust in your data and make better decisions.
Summary
In summary, data reliability is the foundation of accurate and consistent data over time. Unreliable data can lead to costly decision-making mistakes, while reliable data can provide a competitive edge. By understanding and measuring data reliability,you can maintain the accuracy and consistency of your data and make better decisions.
Table of contents
Tags
...

...

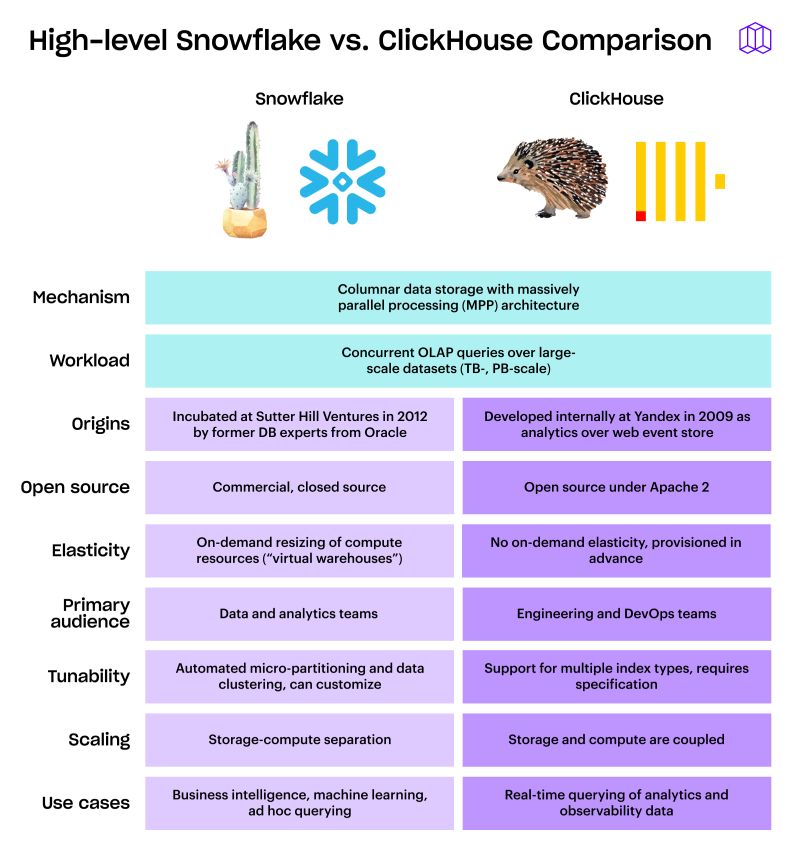


