How Ramp uses data
Learn how giving data a seat at the table has powered the growth of one of the world's most successful fintech companies.
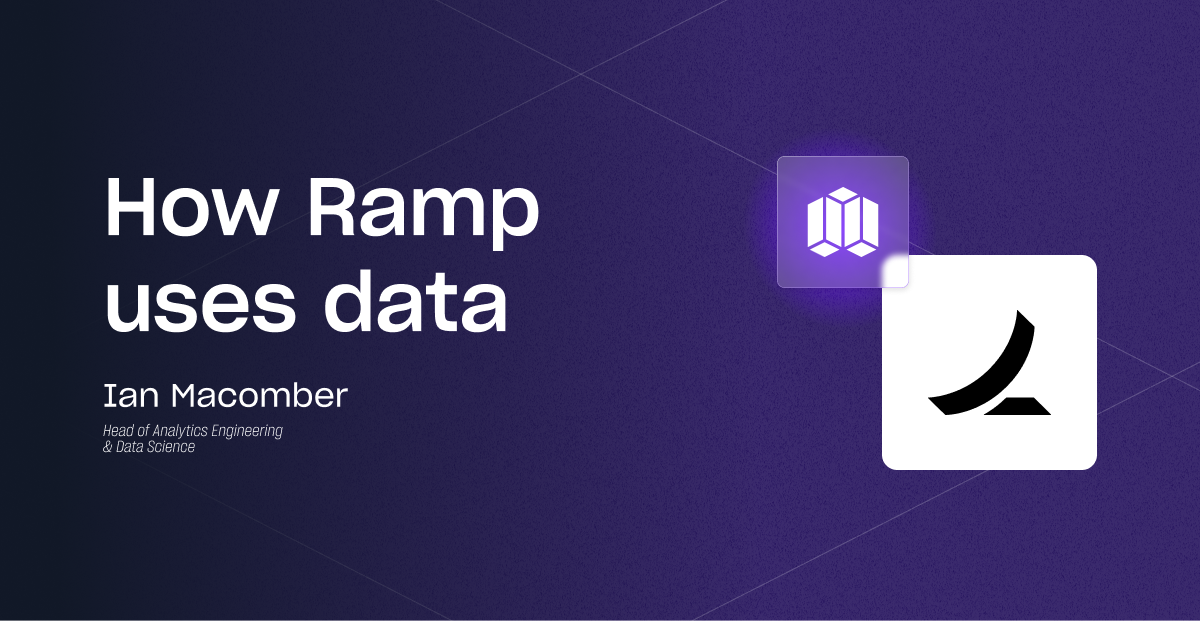
You hear the saying all the time that "data is a company’s only true proprietary asset."
At the same time, it can feel like data teams are pretty far removed from the room where it happens. They might make dashboards for the people in the room, but data practitioners are rarely the ones informing the roadmap and driving innovation at a company.
That’s certainly not the case at Ramp. By utilizing data as a core asset and differentiator, the company hit $100 million in revenue in its first two years, recently raised a $150 million funding round at a $7.65 billion valuation, and became widely recognized as one of the leading fintech companies in the world.
In an interview with Ian Macomber, Head of Analytics Engineering and Data Science at Ramp, we learn how Ramp leverages data to gain a competitive edge, how it’s productized to benefit Ramp users, and how Ramp’s approach to data helps make it all happen.
Starting with core capabilities
A large part of how Ramp spent their first couple of years was building feature parity with their competitors—albeit all under one roof.
This was true of their data team as well. Rather than trying to build data use cases across the entire company, Ian and his team focused on one specific use case at a time.
Early on, that looked like applying data analytics solutions to the risk and fraud portion of the business. It’s not the most exciting or innovative part of what Ramp does, but when banks and other companies have real money at stake, it’s arguably the most critical to get right.
“We chose to underinvest in other parts of the business so that we could have really great success on risk, compound some wins there, then pull our heads up and figure out what the next surface area is,” says Ian.
This approach led to really strong foundations and set the data team up to go after more ambitious, forward-looking projects.
Turning data into a competitive advantage
Once the basics were established, Ian and his team were able to start thinking about how to productize the massive loads of anonymized data that they had.
A recent example of this is Ramp’s Price Intelligence tool. Price Intelligence uses spend data from thousands of Ramp customers and millions of Ramp transactions to help customers understand whether they’re paying too much for software.
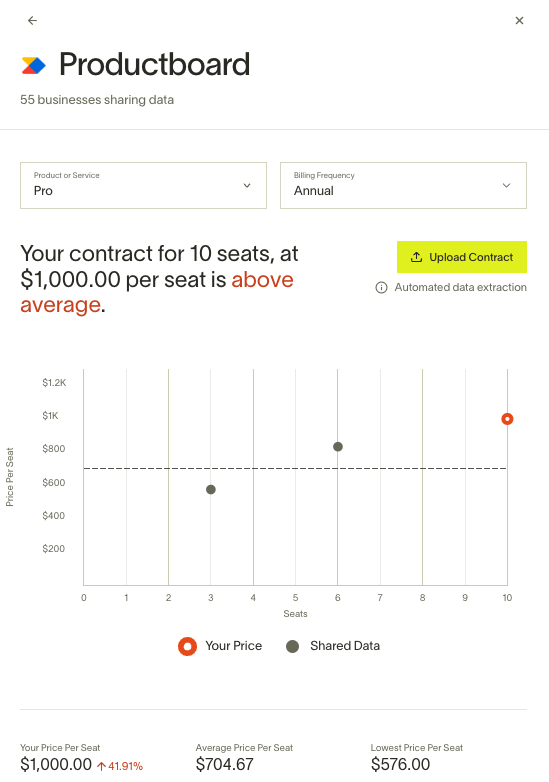
How do they do it? They started by determining what factors went into the final cost of a particular software on each invoice—knowing this is what’s important to parse out. Then, they extracted that unstructured data, turned it into structured data, and moved it into Snowflake for their data team to work with. Then, they leverage LLMs to figure out what, on average, is a fair price for each SaaS vendor.
Leveraging their unique, proprietary data, the Ramp team was able to ship a service that many companies would probably pay for as a stand-alone product.
Structure of Ramp’s data team
Quite famously, Ramp’s data team works in pods. You can think of them as small, cross-functional teams comprising folks from product, engineering, design, and data.
Everyone on the data team is grouped into a general area of expertise—like product, growth, risk, etc—but they’re then placed in one to two pods to tackle specific projects.

Data team members are typically placed in pods for six months, minimum, so that they can get embedded with a particular team, gather all the context they need, and then meaningfully contribute to solving the problem.
“They’re not data science support for the accounting pod,” says Ian. “They are the accounting pod.” That distinction has made a meaningful difference in how data informs Ramp’s product roadmap.
Using Metaplane to ensure data quality
As you can imagine, the finance industry is highly regulated. For that reason, it’s pivotal that Ramp’s financial data is correct the first time without any issues.
“Our data comes in a variety of sources, a variety of cleanliness, and a variety of latencies—and it all needs to fit together quite nicely,” says Ian. Metaplane helps his team do that.
For example, Ramp’s data team sends a set of CSV files to their lenders daily. These files contain various data sets, including Gross Liquid Assets (GLA)—which you could loosely define as the ability of Ramp customers to pay back Ramp.
One day, this number dropped 25% overnight—something that, if true, would mean big trouble. Luckily, a Metaplane alert kicked in to let Ramp’s data team know. They quickly did some root cause analysis and saw they had a couple of bank accounts that were overstated by 100x.
“This is not something our testing suite would have caught,” says Ian. Now, instead of alarming their lenders, they were able to proactively let them know about the issue, tell them what went wrong, and give them the accurate information they needed.
This goes a long way in preserving data trust not only with Ramp's lenders, but their own internal teams, too.
If you want to learn more about how to use Metaplane to get more visibility into your data and build more trust, try us for free, or talk to our team.
Table of contents
Tags
...

...

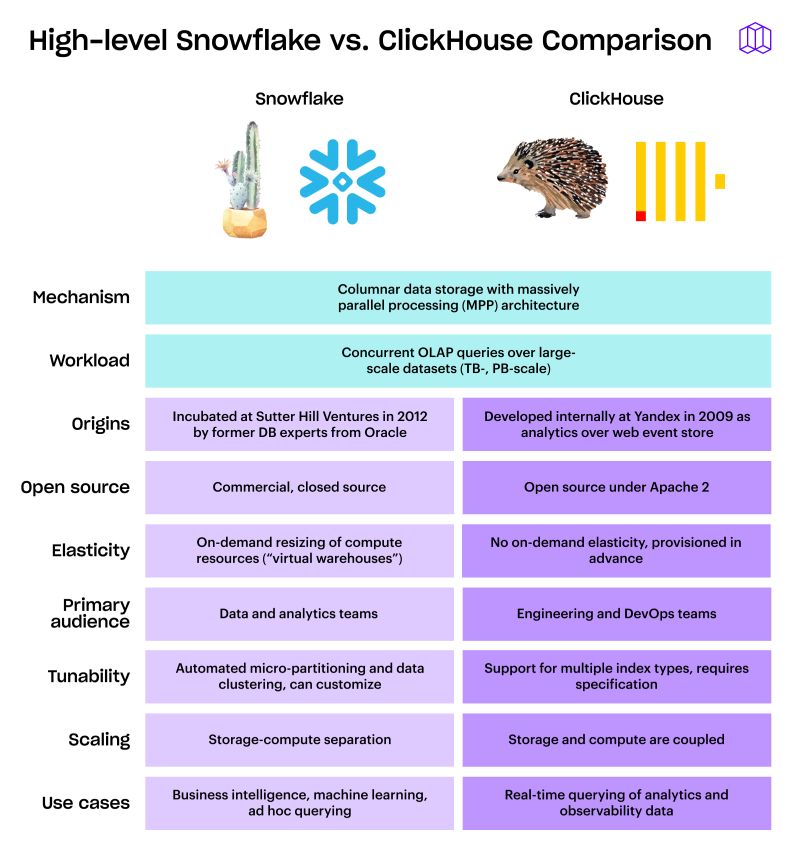


