Machine Learning Observability vs Data Observability
As data becomes a crucial element of decision-making in organizations, observability has become a key requirement. It is essential to ensure that the data is reliable, accurate, and consistent, especially within a modern data stack. In this article, we will explore two important types of observability for modern data teams - machine learning observability and data observability.

What is Data Observability?
Data observability is the practice of monitoring and troubleshooting data pipelines and data infrastructure to ensure that the data flowing through the system is accurate, complete, and consistent. It involves measuring and tracking data quality metrics such as freshness, completeness, and schema consistency.
In data engineering, data observability is used to monitor data as it moves through the ETL (extract, transform, load) process, data warehouses, and data lakehouses. It helps data engineers and data analysts identify and troubleshoot issues that could lead to inaccurate or unreliable data in downstream analysis and decision-making.
What is Machine Learning Observability?
Machine learning observability is concerned with monitoring and understanding the behavior and performance of machine learning models. It involves monitoring the behavior and performance of entire models or algorithms, including their inputs, outputs, and internal states. Machine learning observability is tailored for data scientists and machine learning engineers who require models that perform optimally to extract insights from their data.

In machine learning, observability is crucial for identifying and troubleshooting issues with model behavior and performance, ensuring that the models perform as expected, and improving their accuracy and effectiveness over time. It helps data scientists and machine learning engineers monitor and improve the performance of models deployed in production settings.
How do Data Observability Tools use Machine Learning?
Data observability typically leverages machine learning and metadata to train models and update data quality tests automatically. These tools can account for new patterns and anomalies in the data and automatically adjust data quality tests. By leveraging machine learning to automate data quality monitoring, data engineering teams can spend more time on value-adding activities and less time on maintaining pipelines.
Here are three examples of when data quality tests need to be updated by machine learning:
- The underlying data source changes.
- The data distribution changes.
- New anomalies, patterns, or changes appear in the data.
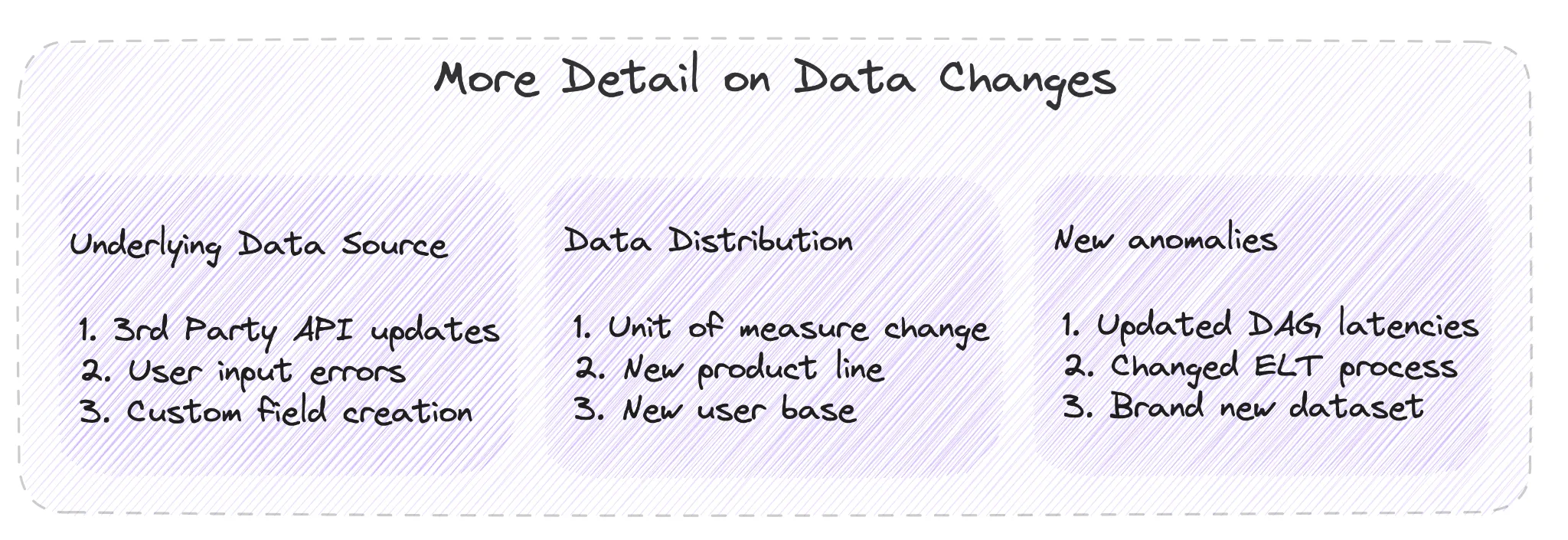
Metaplane offers such data observability tools, designed for the modern data stack. They provide monitoring and troubleshooting tools to ensure data quality. With our platform, data teams can monitor the data pipeline, ETL operations, and data warehouse in real-time. In addition, our platform is user-friendly, making it easy to identify and fix data issues without requiring coding expertise.
Data Observability vs ML Observability Differences
Data Use Case Focus
Data observability primarily concerns the monitoring and quality assurance of data pipelines and data infrastructure, while machine learning observability focuses on monitoring and understanding the behavior and performance of machine learning models.
Dataset Focus
Data observability is concerned with monitoring the flow and characteristics of individual data points, ensuring data integrity, consistency, and accuracy. Machine learning observability focuses on monitoring the behavior and performance of entire models or algorithms, including their inputs, outputs, and internal states.
Metrics and Signals
Data observability relies on metrics and signals related to data quality, such as data freshness, completeness, and schema consistency. Machine learning observability involves metrics and signals that assess model performance, such as accuracy, precision, recall, and various evaluation metrics.
Techniques and Features
Data observability employs tools and techniques like data profiling, data lineage tracking, and anomaly detection to ensure data quality. Machine learning observability utilizes techniques like model performance monitoring, feature importance analysis, and model explainability to gain insights into model behavior and performance.
Stakeholders and Goals
Data observability primarily benefits data engineers, data scientists, and data analysts who rely on accurate and reliable data for their work. Machine learning observability is crucial for machine learning engineers, data scientists, and business stakeholders to ensure the performance, fairness, and interpretability of machine learning models in production.
Data Observability vs ML Observability Similarities
Ongoing Data Monitoring
Both data observability and machine learning observability require continuous monitoring to ensure the quality and performance of the data pipelines and machine learning models.
Metrics Monitoring
Both disciplines rely on the use of metrics to measure the performance and behavior of the system. They involve monitoring relevant metrics and tracking their changes over time to identify issues, anomalies, or improvements.
Data Validation
Both disciplines involve data validation. Data observability ensures the integrity and quality of data through validation techniques, while machine learning observability validates the inputs and outputs of the models to ensure they align with expectations.
Anomaly Detection
Both disciplines utilize anomaly detection techniques. Data observability looks for anomalies or deviations in data patterns or distributions, while machine learning observability detects anomalies in the behavior or performance of machine learning models.
Root Cause Analysis
Both data observability and machine learning observability aim to identify the root causes of issues or problems in their respective domains. They involve analyzing data and system behavior to understand the underlying reasons for data quality issues or model performance degradation.
Summary
In summary, observability is vital for modern data teams, encompassing both data observability and machine learning observability. The two use cases may intersect with targeted marketing or predicting revenue data. Whereas data observability is primarily concerned with the monitoring and quality assurance of data pipelines, machine learning observability focuses on monitoring and understanding machine learning models' behavior and performance. Overall, both data observability and machine learning observability require continuous monitoring and utilize metrics monitoring and anomaly detection techniques.
Table of contents
Tags
...

...

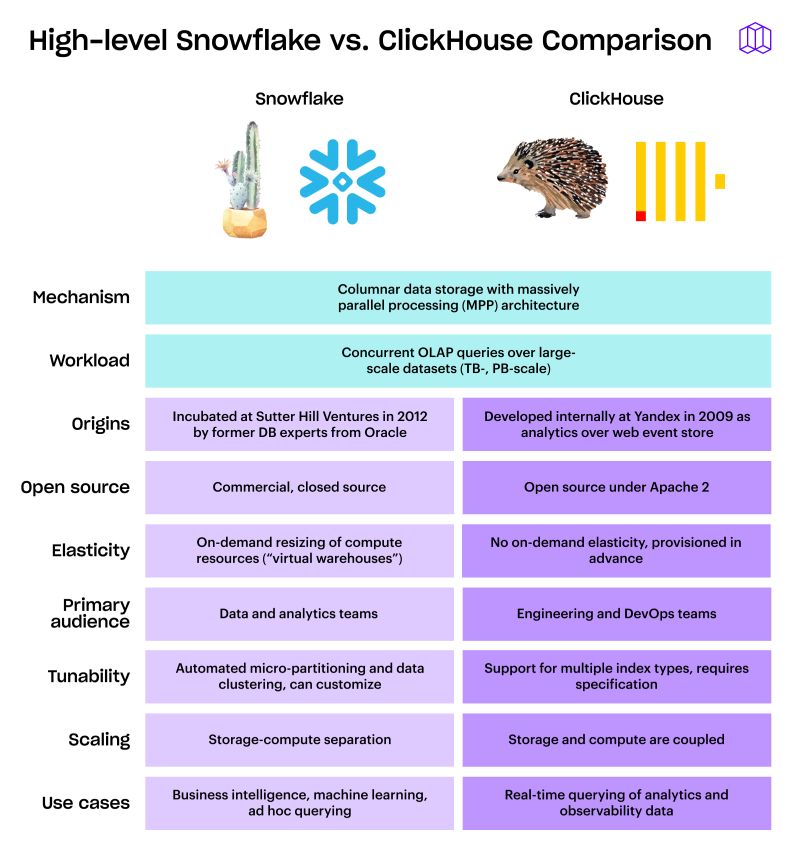


