Proxy and Practicality
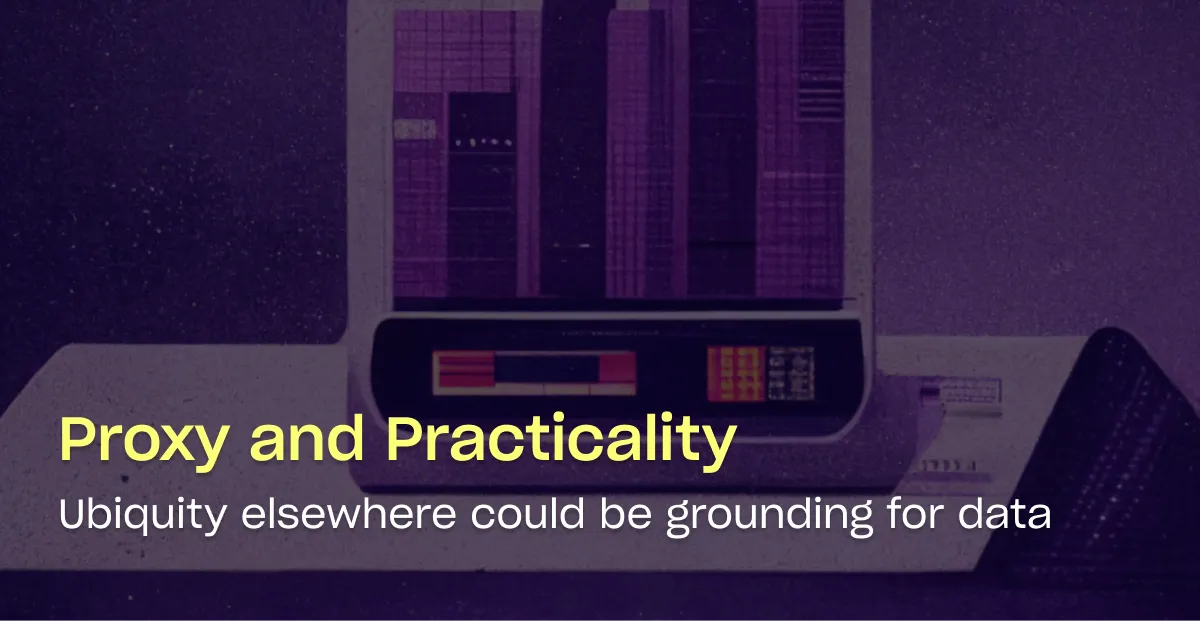
Jason's post on AE roundup inspired so many ideas that they have overflowed into a post.
Proxy
I'm going to try something unusual today. I’m going to draw out some connections I’ve been seeing with an entirely different discipline. The goal here is not to say anything about that particular discipline but rather to share potentially non-obvious lessons I personally took away from my time as a practitioner there.
The discipline in question is politics.
I loved Jason's reflection on his time at Data for Progress and how the lessons learnt there apply to working in the data industry.
I think a discipline that is a bit closer to home is Finance. Finance is a discipline that probably goes back to ancient civilisations in many different forms, which initially accounted for resources and then became much closer to what we know today with the invention of double-entry bookkeeping in Italy, between the 13th and 16th centuries. In some ways, you could view double-entry bookkeeping as a form of version control on the data itself.
I recently outed myself as having an accounting qualification 😅. I have never held a true Finance role - always data roles and, for over half of my career, these have been in Finance departments. Understanding both disciplines well, I see big parallels between the roles in the data space today and well-established Finance roles:
Data Engineer :: Finance Consultant - Typically, Finance teams bring in consultants to set up their infrastructure (such as Workday) and to make sure that the necessary information is flowing in and out of these systems. They are specialists in these tools and how they integrate with other things. This is similar to how Data Engineers have specialist skills in setting up data infrastructure including streaming, lakes and EL.
Analytics Engineer :: Financial Controller - FC teams typically work to build the information that the rest of the Finance team and wider company relies on. They do this by incorporating the information from invoices and cash balances to produce journals, ledgers and financial statements that are well-defined and correct. Notice the similarity with the AE role, which is to build data models that the rest of the Data team and company rely on, incorporating multiple data sources to create an accurate and well-defined model of the business. Could you imagine having GAAEP - Generally Accepted Analytics Engineering Principles? I think we're already on the path to this with all of the courses and programmes teaching it, we now have official qualifications to be held, too.
Analysts & Data Scientists :: Financial Planning & Analysis - FP&A teams (and sometimes Decision Support + Finance Business Partners - specialist offshoots from FP&A for large enough teams) consume the information from FC (and data/commercial/operations) to explain what has happened in the past and compare this to expectations (budget, forecast etc). They also then go on to make their informed predictions about the future performance of the business in the form of Budgets and Forecasts. Analysts & Data Scientists fulfil these roles for data, but in a broader and more general context. I have long thought that data teams could have Data Business Partners - this does actually happen today, but it's usually in the form of embedded Analysts, and, as Katie Bauer mentioned in a recent AE podcast, they probably need to be senior to do this role well. They are not often hired at the right level. Finance Business Partners are at the level of Staff or Principal Data Professionals - they are individual contributors who are at the level of team leads, who would report into a CFO.

I've covered the roles but at a discipline level, the purpose of Finance is to accurately record what has happened to a company's money and assets, to explain what has happened already and to predict and scenario model what will happen in the future. All of these tasks support their organisation to make better decisions. This is so similar to what data does that we can look to their more mature models of influence and support in a business context, which we can copy. We still should look to Software Engineering when we're doing engineering work in data, but for how we should fit into a company from a people and process perspective, Finance is a good forerunner.
Practicality
"While we’re swept up in the 19th iteration of the `is self serve viable` or what an `insight` is - we need to remember these aren’t the things practitioners are thinking about day to day.
There are two main reasons it’s important to recognize this.
1. We can’t Deliver Value without knowing what people actually care about and there are broad swathes of voters data practitioners that aren’t involved in many of the conversation we have. This is why the DX team at dbt Labs has heard me say enough times that they’re quite sick of it that we need to “focus on the kitchen tables issues that matter to your hardworking, everyday analytics engineer”. Things like how to upgrade dbt versions without losing your mind and how to structure your projects.
2. It can be incredibly difficult if you are someone who is newer to The Discourse to get involved. This is really bad! We need to create an environment where if this is your first month or third decade working in data, you feel like the broader data community is here for you. When we get bogged down in the long running and sometimes insular meta-narratives it makes it much harder for folks to get involved. This is top of mind as it was recently raised by an awesome newer member of the data community.
The best way to combat an insular mindset is to make contact with reality."
I must admit to being guilty of spending a lot of time in the meta-narratives of the day, whether that be self-serve, data role history, data app marketplaces, HTAP, streaming... you name it, I've probably waded in. I do agree that a balance between the problems on the ground and the overarching long-term strategy of data architecture and practices is needed. I'm enjoying building Metaplane's data stack and writing about my experience getting my hands dirty, dealing with practical problems.
This is one of the reasons I'm glad to be at Metaplane. We aren't solving an abstract problem, which working on something like a metrics layer can be. Everyone working in data needs to care about Data Quality. Data Observability tools are part of necessary steps to maximise Data Quality. I firmly believe that anyone with a Data Warehouse should have tooling to perform Data Observability (whether that's Metaplane or otherwise), in the same way that no CTO would dream of having a tech stack without one of New Relic/DataDog/Splunk in place, or possibly more than one.

Most data practitioners have experienced Data Shame - where a stakeholder has told you your data is wrong before you knew there was an issue. I'm sure you can remember what it's like - it's a sinking feeling when the message comes in from your stakeholder with the BI link, then there's the panic as you scramble around trying to find out what's wrong in your haystack, the calculation as to how much of your credibility has been eroded… How can they trust our analyses when we can’t get the “basics” right!
This is why, as a Data team lead, I brought in a Data Observability tool. I don't think I'll ever have a heavily used stack that doesn't have one in place again. Avoiding Data Shame = Maximising Data Quality, is a topic that ALL data practitioners care about, whether they are just through the overton window or so far past the overton door that it's a speck on the horizon.
I think this is why in these difficult times, VCs are more interested in funding lower down the stack - they want to fund products that are relevant solutions to a wider audience (also known as TAM in VC terminology). They, rightly, have assessed that Data Quality and general data infra is a problem affecting many organisations, and want to fund in the unsolved parts of this space (eg EL tooling is beginning to feel solved with Fivetran/Airbyte/Rivery/Keboola etc covering most of the surface area and Portable covering the long tail).
During a downturn, it's natural to think that most data teams will focus on getting their fundamentals right, using limited resources. Organisations that run on data will still need it to work and be correct, even under stress. They may decide that now is not the time to look at more abstract or advanced data tooling in this current climate, unless it can save them money elsewhere (buy Continual vs hire a $1m USD DS team?)
Table of contents
Tags
...

...

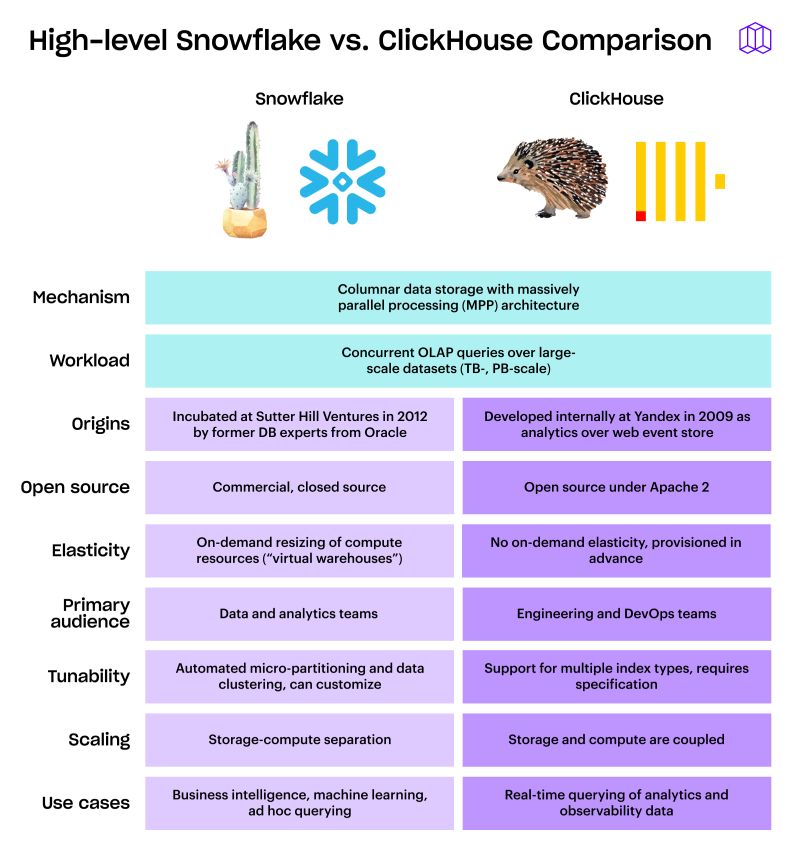


