Snowflake MERGE: The do-it-all command for data manipulation
Learn what the MERGE statement does Snowflake and examples and best practices for using it.

Data rarely stands still. As a data engineer, you're constantly dealing with streams of updates, inserts, and deletions, and finding efficient ways to manage these changes is essential for maintaining accurate and performant data systems.
The Snowflake `MERGE` statement offers a powerful solution to this challenge by combining `INSERT`, `UPDATE`, and `DELETE` operations into a single atomic transaction. `MERGE` streamlines what would otherwise require multiple separate operations, reducing complexity and potential for errors. Whether you're implementing slowly changing dimensions, synchronizing data between systems, or performing delta loads, `MERGE` gives you a comprehensive solution for data manipulation.
In this post, we'll explore what the `MERGE` function does, when you might use it, how it compares to other data manipulation approaches, and share some pro tips to level up your data engineering skills.
What is the MERGE function in Snowflake?
The `MERGE` function in Snowflake is a SQL command that lets you simultaneously perform multiple DML operations (`INSERT`, `UPDATE`, `DELETE`) on a target table based on the results of a join with a source table or subquery. `MERGE` compares records in two datasets and applies changes based on conditions you specify, all in one atomic transaction.
Think of it like reconciling your personal budget with your bank statement—you're matching transactions, adding missing ones, updating incorrect ones, and maybe removing duplicates, all in one efficient process.
Basic syntax for MERGE
```sql
MERGE INTO target_table AS target
USING source_table AS source
ON merge_condition
WHEN MATCHED THEN
UPDATE SET column1 = source.column1, column2 = source.column2, ...
WHEN NOT MATCHED THEN
INSERT (column1, column2, ...) VALUES (source.column1, source.column2, ...)
WHEN MATCHED AND delete_condition THEN
DELETE
```
Let's see it in action with a simple example. Say you're managing a customer database and need to update existing customer information while adding new customers:
```sql
MERGE INTO customers AS target
USING customer_updates AS source
ON target.customer_id = source.customer_id
WHEN MATCHED THEN
UPDATE SET
email = source.email,
phone = source.phone,
last_updated = CURRENT_TIMESTAMP()
WHEN NOT MATCHED THEN
INSERT (customer_id, first_name, last_name, email, phone, created_at, last_updated)
VALUES (source.customer_id, source.first_name, source.last_name, source.email,
source.phone, CURRENT_TIMESTAMP(), CURRENT_TIMESTAMP());
```
This query compares records in the `customer_updates` source table with the `customers` target table. If a customer already exists (matched on `customer_id`), it updates their contact information. If the customer doesn't exist yet, it inserts a new record.
When to use MERGE in Snowflake
The `MERGE` function is versatile and widely used. Let's explore some common ways data engineers leverage it in Snowflake.
1. Implementing slowly changing dimensions (SCDs)
Slowly Changing Dimensions are a cornerstone concept in data warehousing, where you need to track historical changes to dimension data over time. `MERGE` makes implementing Type 1 (overwrite) and Type 2 (historical versioning) SCDs much simpler.
Imagine you're maintaining a product dimension table for an e-commerce platform, and you need to update product information while tracking historical changes:
```sql
MERGE INTO dim_products AS target
USING product_updates AS source
ON target.product_id = source.product_id AND target.is_current = TRUE
WHEN MATCHED AND (
target.product_name != source.product_name OR
target.category != source.category OR
target.price != source.price
) THEN
UPDATE SET
is_current = FALSE,
end_date = CURRENT_DATE()
WHEN NOT MATCHED THEN
INSERT (product_id, product_name, category, price, start_date, end_date, is_current)
VALUES (
source.product_id,
source.product_name,
source.category,
source.price,
CURRENT_DATE(),
NULL,
TRUE
);
-- Insert new versions for updated products
INSERT INTO dim_products (
product_id, product_name, category, price, start_date, end_date, is_current
)
SELECT
source.product_id,
source.product_name,
source.category,
source.price,
CURRENT_DATE(),
NULL,
TRUE
FROM product_updates AS source
JOIN dim_products AS target
ON target.product_id = source.product_id
AND target.is_current = FALSE
AND target.end_date = CURRENT_DATE();
```
This approach implements a Type 2 SCD pattern where:
- The `MERGE` statement marks existing records as no longer current when changes are detected
- The following `INSERT` creates new "current" records with updated information
- Historical versions are preserved with their valid date ranges
2. Syncing data between systems
When integrating data between different systems, `MERGE` provides an elegant way to keep destination tables in sync with their sources.
Imagine your marketing team uses a third-party platform with campaign data that you need to sync daily with your data warehouse:
```sql
MERGE INTO marketing_campaigns AS target
USING staging_campaigns AS source
ON target.campaign_id = source.campaign_id
WHEN MATCHED AND source.is_deleted = TRUE THEN
DELETE
WHEN MATCHED THEN
UPDATE SET
campaign_name = source.campaign_name,
channel = source.channel,
budget = source.budget,
start_date = source.start_date,
end_date = source.end_date,
last_updated = CURRENT_TIMESTAMP()
WHEN NOT MATCHED THEN
INSERT (campaign_id, campaign_name, channel, budget, start_date, end_date, created_at, last_updated)
VALUES (
source.campaign_id,
source.campaign_name,
source.channel,
source.budget,
source.start_date,
source.end_date,
CURRENT_TIMESTAMP(),
CURRENT_TIMESTAMP()
);
```
This query handles all three common synchronization scenarios:
- Updates existing campaign information
- Adds new campaigns that weren't previously tracked
- Removes campaigns that have been deleted in the source system
3. Incremental loading/delta processing
One of the most common data engineering tasks is efficiently loading incremental changes without duplicating or missing records. `MERGE` makes this pattern relatively straightforward:
```sql
MERGE INTO sales_facts AS target
USING (
SELECT * FROM staging_sales
WHERE transaction_date >= DATEADD(day, -1, CURRENT_DATE())
) AS source
ON target.transaction_id = source.transaction_id
WHEN MATCHED THEN
UPDATE SET
amount = source.amount,
quantity = source.quantity,
last_updated = CURRENT_TIMESTAMP()
WHEN NOT MATCHED THEN
INSERT (transaction_id, product_id, customer_id, store_id, transaction_date, amount, quantity, created_at, last_updated)
VALUES (
source.transaction_id,
source.product_id,
source.customer_id,
source.store_id,
source.transaction_date,
source.amount,
source.quantity,
CURRENT_TIMESTAMP(),
CURRENT_TIMESTAMP()
);
```
This query processes only recent transactions (from the last day), which:
- Significantly reduces the processing overhead compared to full reloads
- Updates any records that may have been modified
- Inserts only new transactions
- Maintains data consistency in a single atomic operation
4. Data deduplication and cleansing
`MERGE` can be particularly useful for cleaning up data with duplicates or inconsistencies.
Imagine you have multiple sources feeding customer information, resulting in duplicate records that need to be consolidated:
```sql
-- Create a temp table with the "golden record" for each customer
CREATE TEMPORARY TABLE customer_golden_records AS
SELECT
customer_email,
MAX(customer_id) AS customer_id,
COALESCE(MAX(NULLIF(first_name, '')), 'Unknown') AS first_name,
COALESCE(MAX(NULLIF(last_name, '')), 'Unknown') AS last_name,
COALESCE(MAX(NULLIF(phone, '')), 'N/A') AS phone,
MAX(last_updated) AS last_updated
FROM customers_raw
GROUP BY customer_email;
-- Use MERGE to deduplicate and clean the customer table
MERGE INTO customers AS target
USING customer_golden_records AS source
ON target.customer_email = source.customer_email
WHEN MATCHED AND target.customer_id != source.customer_id THEN
DELETE
WHEN MATCHED THEN
UPDATE SET
first_name = source.first_name,
last_name = source.last_name,
phone = source.phone,
last_updated = CURRENT_TIMESTAMP()
WHEN NOT MATCHED THEN
INSERT (customer_id, customer_email, first_name, last_name, phone, created_at, last_updated)
VALUES (
source.customer_id,
source.customer_email,
source.first_name,
source.last_name,
source.phone,
CURRENT_TIMESTAMP(),
CURRENT_TIMESTAMP()
);
```
This approach first creates a "golden record" for each customer based on their email address, then uses `MERGE` to:
- Remove duplicates (by deleting records with non-preferred IDs)
- Update existing customer records with the most complete information
- Add new customers who don't yet exist in the target table
MERGE vs. other data manipulation approaches
While `MERGE` is powerful, it's not the only way to manipulate data in Snowflake. Let's compare it to other common approaches:
Key benefits of MERGE
- Atomicity: All operations succeed or fail together, maintaining data integrity
- Performance: Often more efficient than separate DML statements
- Readability: Consolidates complex logic into a more readable format
- Traceability: Easier to track all changes in a single transaction
Tips and tricks when using MERGE in Snowflake
To get the most out of `MERGE`, keep these best practices in mind:
1. Optimize join conditions
The `ON` clause in your `MERGE` statement is critical for performance. Use it to join on indexed or unique columns when possible:
```sql
-- Good: Merging on primary key
MERGE INTO customers AS target
USING updates AS source
ON target.customer_id = source.customer_id -- customer_id is the primary key
-- Less Efficient: Merging on non-indexed columns
MERGE INTO customers AS target
USING updates AS source
ON target.email = source.email AND target.phone = source.phone
```
2. Limit source data volume
Processing only the data you need in the source query can dramatically improve performance:
```sql
-- Process only recent data
MERGE INTO facts AS target
USING (
SELECT * FROM staging_facts
WHERE load_date = CURRENT_DATE() -- Filter in the source query
) AS source
ON target.event_id = source.event_id
WHEN MATCHED...
```
3. Use MERGE conditionally
You can add additional conditions to each `WHEN` clause for more precise control:
```sql
MERGE INTO products AS target
USING updates AS source
ON target.product_id = source.product_id
WHEN MATCHED AND source.price != target.price THEN
UPDATE SET price = source.price, last_updated = CURRENT_TIMESTAMP()
WHEN MATCHED AND source.is_discontinued = TRUE THEN
DELETE
WHEN NOT MATCHED AND source.price > 0 THEN
INSERT...
```
4. Handle constraints carefully
When your target table has constraints (unique, foreign keys, etc.), consider how `MERGE` might impact them:
```sql
-- Use MATCHED clauses in the right order to avoid constraint violations
MERGE INTO employees AS target
USING updates AS source
ON target.employee_id = source.employee_id
WHEN MATCHED AND source.status = 'TERMINATED' THEN
DELETE -- Process terminations first to avoid constraint errors
WHEN MATCHED THEN
UPDATE...
WHEN NOT MATCHED THEN
INSERT...
```
5. Consider transaction size
For very large operations, consider breaking the `MERGE` into smaller batches to avoid long-running transactions:
```sql
-- Process one day at a time for a historical load
DO
$$
DECLARE
current_date DATE := '2023-01-01';
end_date DATE := '2023-12-31';
BEGIN
WHILE current_date <= end_date LOOP
MERGE INTO target AS t
USING (SELECT * FROM source WHERE date = current_date) AS s
ON t.id = s.id
WHEN MATCHED THEN...;
current_date := DATEADD(day, 1, current_date);
END LOOP;
END;
$$;
```
Master MERGE for efficient data operations
The `MERGE` statement is one of the most versatile tools in your SQL toolkit. It combines the power of multiple DML operations into a single, atomic transaction that's both easier to maintain and often more efficient to execute.
Whether you're implementing slowly changing dimensions, synchronizing data between systems, processing incremental loads, or cleaning messy data, `MERGE` offers a clean, declarative approach that scales with your data operations.
As you incorporate `MERGE` into your data pipelines, remember to optimize your join conditions, limit source data volumes, and be mindful of transaction sizes for the best performance. With these best practices in mind, you'll be well-equipped to handle complex data manipulation tasks with confidence and efficiency.
Now, the next time you're faced with the puzzle of efficiently updating your Snowflake tables, you'll know exactly which tool to reach for: `MERGE`.
Looking for more Snowflake tips? Learn how to use DATEDIFF, DATE_TRUNC, IFNULL, COALESCE, NVL, and DATEADD.
Table of contents
Tags
...

...

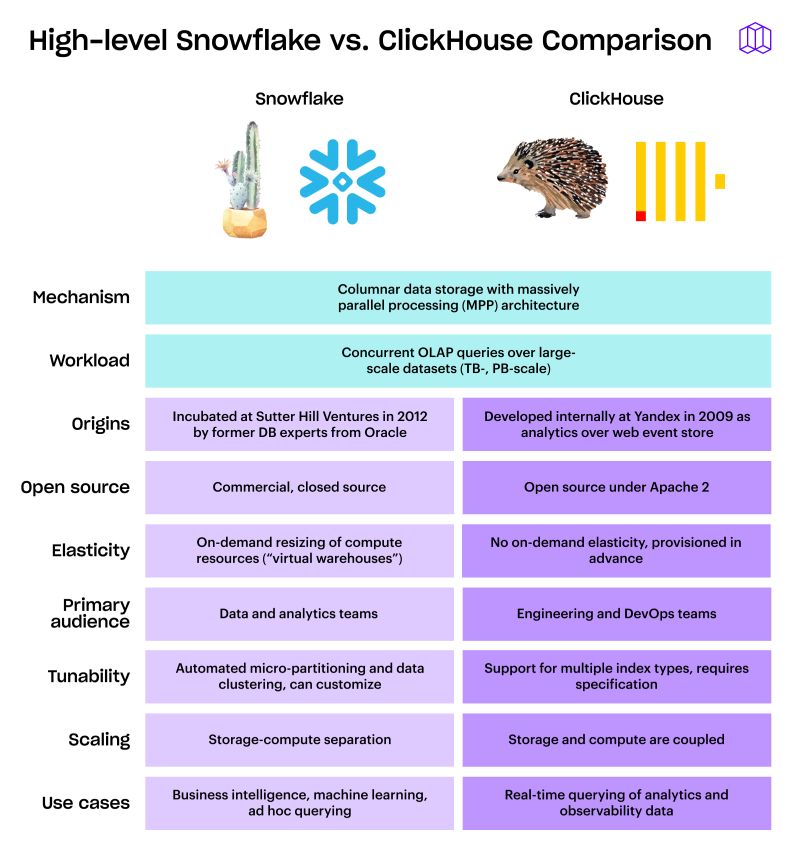


