The Role of Data Observability in Data Governance Policies
Your sales department wants to automate the e-mail campaigns, but you're unsure whether your data governance policy permits it. How can you know enough to make the right decision? As the data industry becomes more regulated and monitored, data observability has a crucial role in ensuring compliance with data governance policies. In this post, we’ll examine the significance of data governance policy for organizations, the definition of data observability and its role in data governance, achieving data observability in organizations, and real-world data observability and data governance examples.

Understanding Data Observability
Data observability refers to the ability to understand what is happening within a software system or application based on its outputs. Data observability tools such as Metaplane make this possible by allowing data teams to monitor, troubleshoot and ensure data quality across their stack. Implementing data observability tools adds transparency, reliability, and integrity, enabling data teams to adapt and evolve sustainably.
The benefits of implementing data observability tools are numerous. For one, data teams can catch data pipeline anomalies and detect how they happened by obtaining historical records of when and how they were deployed. Additionally, data observability tools make it possible to monitor data quality and maintain data integrity, ensuring that organizations can trust their data and make more informed business decisions. Perhaps most importantly, data observability and transparency are inherently interconnected, and observability is critical to promoting transparency in complex systems.
The Significance of Data Governance Policy for Organizations
Data governance refers to the process of managing the integrity, availability, and security of data used in an organization. A data governance policy is critical for modern data-driven organizations as a means of ensuring that they can effectively manage, understand, and utilize their data.
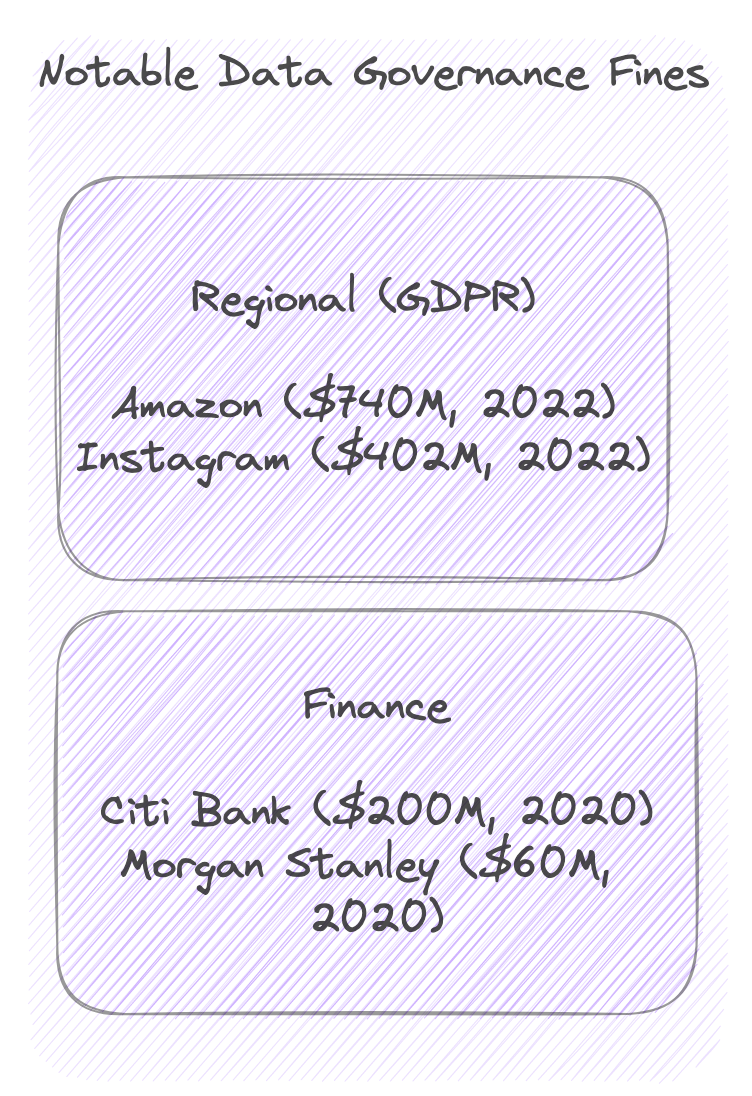
A data governance policy is necessary for several reasons, such as regulatory fines, loss of brand reputation, and legal repercussions. For example, the European Union's General Data Protection Regulation (GDPR) protects European citizens' personal data by regulating how personal data is collected, processed, used, or shared. A data governance policy is critical for effective data protection.
Data governance policy evolves depending on the industry and region. For example, industries that handle personally identifiable information (PII) may have tighter regulatory restrictions than those that do not. It is essential to understand the specific regulations governing an organization's data and to have a data governance policy that complies with those regulations.
The Intersection of Data Governance and Data Observability
Data observability can enhance the effectiveness of a data governance policy by ensuring that data quality is high, increasing the change that regulatory requirements are complied with, and cybersecurity risks minimized. Additionally, data teams can use data observability to enhance their understanding of data usage, thereby increasing their ability to detect misuse, malfeasance, or potential regulatory infractions.
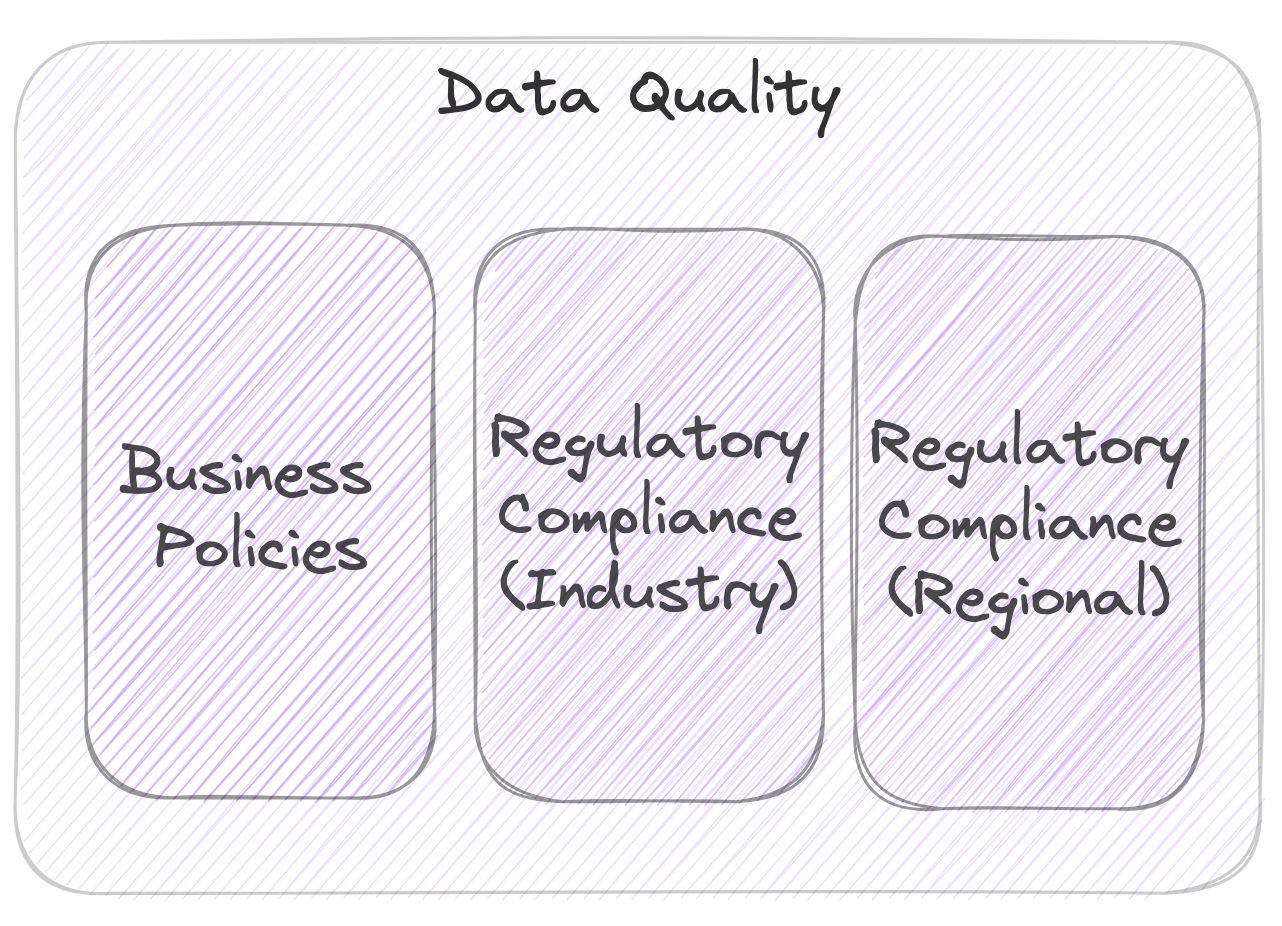
By aligning a company's data observability with its data governance policy, organizations can build a transparent data culture that is protective of organizational assets, compliant with regulatory requirements, and inherently open to collaboration.
Approximating Data Observability in Organizations
It's possible to elevate your data quality tests to accomplish much what Data Observability tools serve to do. The main gaps that we've seen between home grown data quality tests and data observability tools, when it comes to detecting data quality issues, is primarily: speed of deployment, test type coverage, and test acceptance threshold calculations. Secondary to that is the ability to build lineage, to help you monitor user access to sensitive datasets.
In this case, the most important data quality tests, when it comes to finding PII, includes:
- Freshness: This leading indicator helps you to understand whether pipelines are running as expected, including those handling user information.
- Row counts: This is a secondary indicator that helps you to identify whether pipelines are working with their expected volume of data.
- String formatting: If you're using something like email address as the unique identifier for an individual - you may want to make sure that all emails are entered properly.
- Referential integrity: In cases of 1:N or N:1 relationships, simply using row count isn't enough. Instead, you may want to make sure that you're able to locate a singular user across multiple tables, through their unique user id (or similar).
Examples of Using Data Observability for Data Governance
For our first example, let’s say that you’re Head of Data at Rainforest, the e-commerce company that sells hydroponic aquariums to high-end restaurants. One of your tables is activated_users, which captures user e-mail data. Suppose you want your sales team to use that table to send marketing e-mails but are unsure if your data governance policy permits it. By using data observability, you can identify possible risks, monitor data quality, and guarantee data integrity.
Another example is handling a data purge request by an individual. In this case, this individual is governed by GDPR and has invoked the "right to be forgotten", which, in this case, means purging their information from our Rainforest user database. If you're able to ensure data quality throughout your pipeline, it'll be much easier to join tables and programmatically delete user information across different datasets.
Summary
Organizations today must deal with ever-shifting data governance paradigms and respond to changing regulatory needs and best practices. Data observability can play an integral and critical role in effective data governance policies that add transparency, reliability, and integrity to the data stack.
To achieve sustainable and trustworthy data observability, data teams should use reliable data observability tools such as Metaplane and follow best practices for implementing data observability in the context of a data governance policy. With proper alignment between data observability and data governance, sales leaders can make better decisions, improve machine learning services, and automate marketing emails with reverse ETL.
Table of contents
Tags
...

...

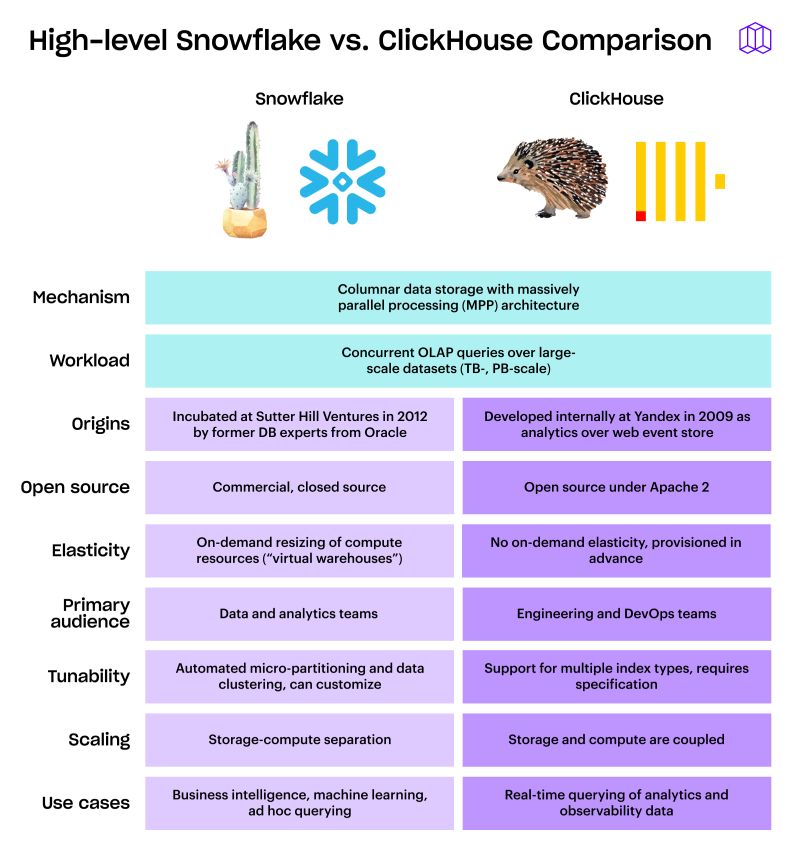


