Data Mesh vs. Data Fabric: Key Differences and Which to Choose in 2023
As data remains the lifeblood of organizations in 2023, the challenge of effectively managing, processing, and storing this data becomes a primary concern. Among the popular choices that have emerged for this purpose are the Data Mesh and Data Fabric architectures. Both of these paradigms present their unique benefits and drawbacks, and determining the right fit for your organization necessitates thoughtful deliberation.
In this post, we'll dissect the main differences between these two architectures and guide you to decide which one suits your organizational needs the best.

Introduction
Before we delve into the specifics of Data Mesh and Data Fabric, let's start by understanding what these terms signify.
Data Mesh
Data Mesh, a concept coined and popularized by Zhamak Dehghani, a thought leader in the field of distributed systems at ThoughtWorks, is a novel data platform design paradigm that emphasizes domain-driven decentralized data management, self-serve data infrastructure, and a federated governance model. It devolves ownership and architectural decision-making to autonomous cross-functional teams who manage different business domains, effectively treating data as a product.
Data Fabric
Data Fabric, a term popularized by Microsoft, is an architectural approach that creates a unified data layer across diverse data sources, facilitating easy data access and processing. This unified approach provides a foundation for advanced data management, governance, and data processing. It aims to streamline data integration and enable efficient data operations across an organization's data landscape.
Determining the most suitable architecture for your data platform grows increasingly crucial as data products emerge as key drivers of business strategy.
Understanding Data Mesh
Data Mesh has emerged as an innovative paradigm to address the limitations of traditional monolithic data platforms.
In essence, Data Mesh emphasizes a domain-oriented approach to data management. Data is owned and managed by each domain such as sales, human resources, or operations, where each domain operates its own infrastructure to create, store, and manipulate data. This data can then be consolidated into a federated structure.
The key advantage of a Data Mesh is its ability to scale effectively, particularly when grappling with complex data models. Its decentralized design allows better granularity and ownership of data, which can expedite insights and data-driven decision-making.
However, the implementation of a Data Mesh architecture also brings forth certain challenges, such as an increase in data modeling complexity and potential surge in infrastructural costs. Maintaining high-quality data can also be a hurdle due to the distributed ownership of data, complicating the enforcement of standardization and data governance policies.
This is where the concept of data observability becomes pivotal. Tools like Metaplane's automated data quality checks and change notifications can aid in ensuring high-quality data and visible changes across the entire architecture.
Overall, a Data Mesh architecture can be an optimal choice for organizations dealing with complex data models and requiring increased scalability. Nonetheless, careful planning, high-quality infrastructure, and robust governance policies are prerequisites for its effective implementation.
Understanding Data Fabric
While Data Mesh underlines a decentralized approach to data management, Data Fabric represents a seamless and interconnected architecture that unifies diverse data sources across an organization.
In a Data Fabric setup, data from disparate sources is integrated into a single layer, making the data easily accessible and processable regardless of its location or application.
The benefits of Data Fabric architecture are manifold, including easy data access, improved data integration, enhanced data governance, and scalability. However, the need for a sophisticated data integration and governance mechanism to ensure seamless data flow and high-quality data can be a challenge.
Data observability remains critical in a Data Fabric setup, facilitating easier identification of data inconsistencies and ensuring that data is appropriately managed. Metaplane's tools can assist in monitoring the quality and consistency of your data across the entire architecture.
A Data Fabric architecture is ideally suited for organizations looking to achieve seamless integration of diverse data sources and enhanced data accessibility.
Data Mesh vs Data Fabric: Key Differences
The primary distinction between Data Mesh and Data Fabric architecture lies in their foundational principles. Data Mesh advocates a decentralized approach to data management, while Data Fabric promotes seamless integration of disparate data sources into a single layer.
In a Data Mesh, data is distributed across domains, with each domain accountable for managing its own data infrastructure. This approach fosters better data ownership and governance but can complicate standardization and quality control across domains.
Conversely, Data Fabric aims to create a unified layer of diverse data sources, facilitating easy data access and processing. However, this approach demands sophisticated data integration and governance mechanisms to ensure seamless data flow and maintain high-quality data.
Data Mesh | Data Fabric | |
---|---|---|
Design Principle | Decentralized approach with data divided among different business domains. | Unified approach with data from disparate sources integrated into a single layer. |
Data Management | Each domain owns and manages its own data, potentially increasing scalability and granularity of data. | Data from diverse sources is seamlessly integrated and easily accessible, regardless of its location or application. |
Data Governance | Data governance is distributed, with each domain taking responsibility for its own data quality and standardization. | Requires a sophisticated data integration and governance mechanism to ensure seamless data flow and high-quality data. |
Benefits | Better granularity and ownership of data, potentially leading to faster insights. | Seamless data access, improved data integration, enhanced data governance, and scalability. |
Challenges | Increased complexity in data modeling and potential increase in infrastructure costs. Standardization and data quality can be challenging due to distributed ownership. | Need for a sophisticated data integration and governance mechanism to ensure seamless data flow and high-quality data. |
Factors to Consider When Choosing Between Data Mesh and Data Fabric
Several factors should be weighed when deciding between a Data Mesh and Data Fabric architecture. The complexity of the organization's data models, the requirement for data accessibility, and the existing data infrastructure are key considerations. The choice of the architecture will also greatly depend on whether a decentralized or unified approach to data management aligns better with the organization's goals.
Just as crucial is data observability. With Metaplane's monitoring and troubleshooting tools, you can ensure the high quality and consistency of your data across the entire architecture, no matter which approach you opt for.
Case Studies: Data Mesh and Data Fabric in Action
Let's delve into some real-world examples to illustrate the application of Data Mesh and Data Fabric architectures:
Data Fabric Case Studies
Adobe, a global leader in digital media and marketing solutions, employs a Data Fabric architecture to streamline the integration and processing of its data from diverse sources. This has enabled Adobe to deliver personalized customer experiences based on insights derived from integrated data.
As a relatively new architecture, few other organizations have published their application of a data fabric architecture.
Data Mesh Case Studies
Zalando, a leading online fashion retailer in Europe, has effectively implemented the Data Mesh concept. Managing a complex system comprised of various autonomous teams, each managing their domain, Zalando's move toward a Data Mesh architecture has improved data quality and accelerated decision-making.
Intuit, known for financial software solutions like TurboTax and QuickBooks, has adopted a Data Mesh architecture to efficiently manage their diverse and distributed data sources. The architecture assigns different teams the responsibility to manage their specific data domains, resulting in improved data quality, streamlined workflows, and fostering more productive cross-functional interactions.
Conclusion: Which to Choose – Data Mesh or Data Fabric?
Choosing between a Data Mesh and a Data Fabric architecture is a strategic decision for data leaders. Each approach offers its unique benefits and challenges, and the choice depends on the specific needs of the organization.
Considerations like the complexity of data models, data accessibility requirements, existing data infrastructure, and the organizational preference for a decentralized versus unified approach to data management should influence the decision. Regardless of which architecture is chosen, data observability is a critical element of an efficient data platform.
With Metaplane's data observability platform, you can overcome common data quality challenges and ensure that your data is of high quality and consistent across the entire architecture. To learn more, get started in minutes for free, or book a demo.
As the importance of data in shaping business strategies continues to grow, companies will need adaptable, scalable, and flexible data platforms to cater to their specific needs.
Frequently Asked Questions (FAQs)
Q: What is a Data Mesh architecture?
A: Data Mesh is a novel data platform design paradigm that emphasizes domain-driven decentralized data management, self-serve data infrastructure, and a federated governance model.
Q: What is a Data Fabric architecture?
A: Data Fabric is an architectural approach that creates a unified data layer across diverse data sources, facilitating easy data access and processing.
Q: What are the main differences between Data Mesh and Data Fabric architecture?
A: The primary difference lies in their design principles. Data Mesh emphasizes a decentralized approach to data management, while Data Fabric adopts a unified approach.
Q: What factors should be considered when choosing between Data Mesh and Data Fabric architecture?
A: Factors such as the complexity of data models, data accessibility requirements, existing data infrastructure, and the organization's preference for a decentralized versus unified approach to data management must be considered when deciding between the two approaches.
Q: Why is data observability important in both Data Mesh and Data Fabric architecture?
A: Data observability is crucial in ensuring the quality and consistency of data across the entire architecture, irrespective of the chosen approach.
Q: How does the choice between Data Mesh and Data Fabric architecture impact data governance?
A: In a Data Mesh architecture, data governance is distributed across different domains, with each domain responsible for its own data. In a Data Fabric architecture, there is a need for a sophisticated data integration and governance mechanism to ensure seamless data flow and maintain high-quality data.
Table of contents
Tags
...

...

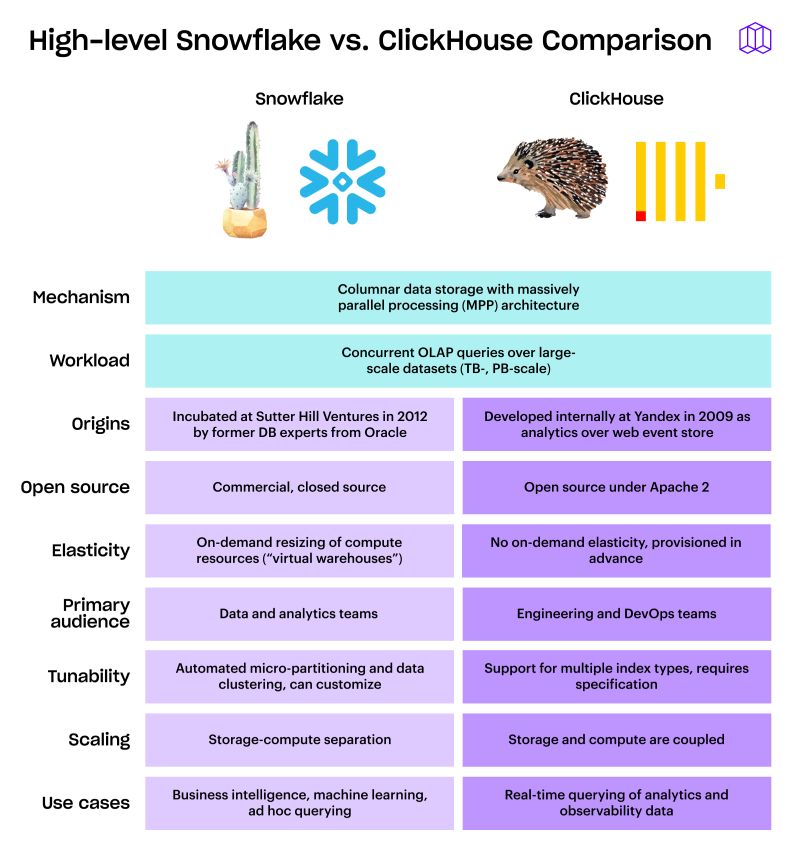


