Data Observability in 10 years
We break down Data Observability into core functional areas of Incident Detection, Triage, and Resolution, and ancillary user experience functions to see how we can project changes that may happen years from now.
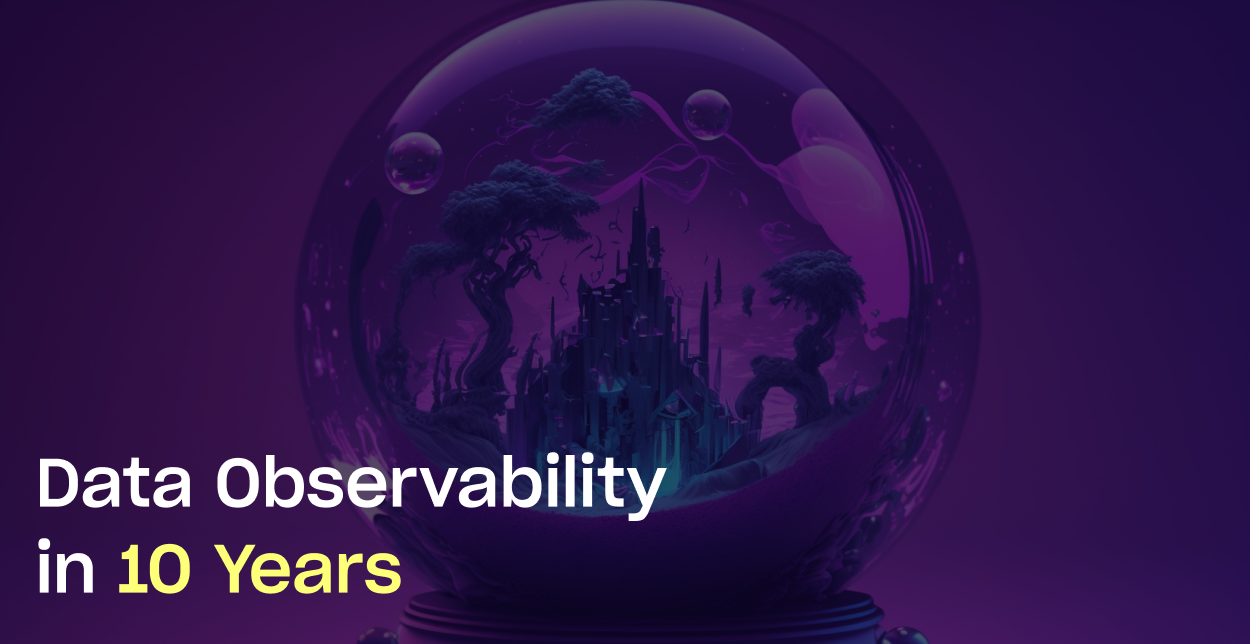
If I could predict the evolution of technology over a long time horizon, I would be on a beach in the Caribbean and not writing this article at 3am in the morning. Drawing inspiration from the Lindy Principle, the practices that survived for 20 years will likely survive for another 20 years. As a result, it’s hard to imagine a future in which SQL, modeling, and relational databases aren't an indispensable part of a data practitioner’s toolkit. Those staples of our diet may have support levels of abstraction on top, like directly manipulating metrics and business objects, but will likely remain constant. Using data observability functionality as a filter for the broader data staples, we can begin to forecast changes seen 10 years from now.
Stable “Jobs to be Done”
When people buy a drill, what they really want is a hole. This is the essence of the “jobs to be done” methodology. Rather than focusing on how an action is performed, and the immediate consequence of what action, the JTBD steps back to ask: what’s the real goal here?
Data Observability as a technology addresses multiple jobs to be done. I think of the jobs in two broad categories: incident management to reduce the frequency and intensity of data issues, and data management that accomplishes use cases with the data at hand, within time/cost/complexity budget.
The incident management job breaks down into four more:
- Incident detection. Be the first to know about potential data incidents.
- Incident triage. Understand probability of real incident, downstream impact, and upstream root cause.
- Incident resolution. Minimize effort and time to resolve data incidents.
- Stakeholder communications. Ensure that consumers of data are aware of its state, and producers of data are aware of their effect on data state.
The data management job has many components, but I’ll focus on:
- Systems integration. Maximize compatibility and minimize siloes between systems.
- Complexity management. Understand and optimize the current and future cost of delivering data to intended use cases.
My prediction is that these jobs aren’t changing any time soon. These jobs have been performed since the advent of the database, although job titles and technologies and trends have shifted over the decades.
Impact to Data Observability
These jobs will stay constant like a buoy in the ocean while the underlying technology and use cases change. Moore's Law will likely continue to bring advancements in storage and compute. As computation becomes cheaper, metadata within systems becomes richer. This rich metadata is surfaced between systems as well, as interchange formats become standardized with time. Alongside these trends, the use cases for data only become more real-time, critical to the business, and expansive across business units.
Considering jobs to be done as the glue between the "demand" of use cases and the "supply" of technologies, we arrive at 10 predictions for Data Observability in 10 years.
Incident Detection
- Observability will be automated. Entities will be monitored based on lineage and frequency of usage, with the types of monitors depending on data types and profiles, frequency of monitors runs depending on update frequency, and confidence intervals based on code changes and related entities.
- Observability will be generic. In addition to capturing metadata like row counts of tables, freshness of views, and lineage between columns, Data Observability systems will be able to collect, store, and monitor any piece of metadata. For example, finding deltas in source-to-target replication, schemas in unstructured events, and data frame sizes in an ML pipeline.
- Shifting left: Extending upstream to verification and validation, interfaces for data producers
- Shifting right: Interfaces for data consumers, business observability
These two predictions maximize monitor coverage and depth while minimizing setup time, cost, and management. But incidents will always happen, leading us to the next logical step, solving for incidents. Starting with answering the triage question of whether an incident is worth devoting resources to.
Incident Triage
- Granular end-to-end lineage: End-to-end column-level lineage from source applications to consumption layer
- Rich usage analytics across the ecosystem: understanding utilization of each tool leveraging metadata within each tool
After triaging an incident and deciding that it requires action, the last step is minimizing time-to-resolve that incident.
Incident resolution
- Root cause analysis: Granular root-cause analysis based on data and code changes
Alongside the core incident management workflows are two core engineering workflows, starting with the perennial problem of siloed system.
Systems integration
- Seamless integration across workflow tools, metadata tools: Imagine an authorization process that doesn't require copying any text fields or certifications over.
But even with integrated systems, the entropy of assets within those systems continue to increase, leading us to...
Complexity management
- Semi-automated optimization of data, code, and assets based on metadata: Usage and query analysis for automated optimization, like deleting unused dashboards
All of these predictions put together lead to one overarching prediction about the "feeling" of data observability in 10 years.
Overarching prediction
- Data Observability becomes a “no-brainer” part of data stacks: Today is like 2013 for software observability. Companies were still early on migration of infrastructure to the cloud.
Bringing on Data Observability in 2033
Imagine you're a new data leader at a startup in 2033. Your company has machine learning and LLMs integrated throughout your product and internal operations. Decisions aren't fully automated, but relevant information is readily available across the company.
Most companies from 2023 have come and gone; your peers only know their names through slide decks at conferences. Their successors are better, with real-time capabilities as needed, built-in validation, deep integrations, and a decade of ergonomics around new interfaces.
Data work mainly involves metrics and business objects now. It still takes work, and many of the challenges like "stakeholder management" and "proving ROI" that the old heads talk about are still real problems.
What has changed, though, is that as a data leader, you have full visibility of all data flows from beginning to end through a Mission Control panel. Transformations, code, and data are all integrated within one application. This data observability platform was a one-click integration across your data store and quickly adapted to your systems.
Most importantly, data producers upstream are aware of the implications of their data changes or inputs, and data consumers downstream are aware of the state of the data they use. Data issues still occur, but they are almost always spotted immediately.
The new formula for data work is: get data, make it work, ensure that it's trusted.
Summary
As someone building a data observability tool, I can't say for sure what tools will be around in 10 years. SQL for sure, the big data warehouses probably, and hopefully Metaplane. But what I am confident about is that the feeling of data work today — one of working in the dark — will be a relic of the past.
Table of contents
Tags
...
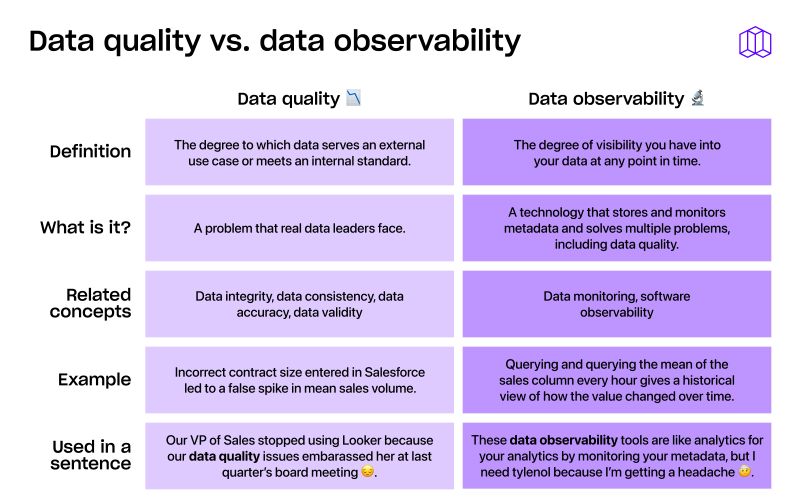
...
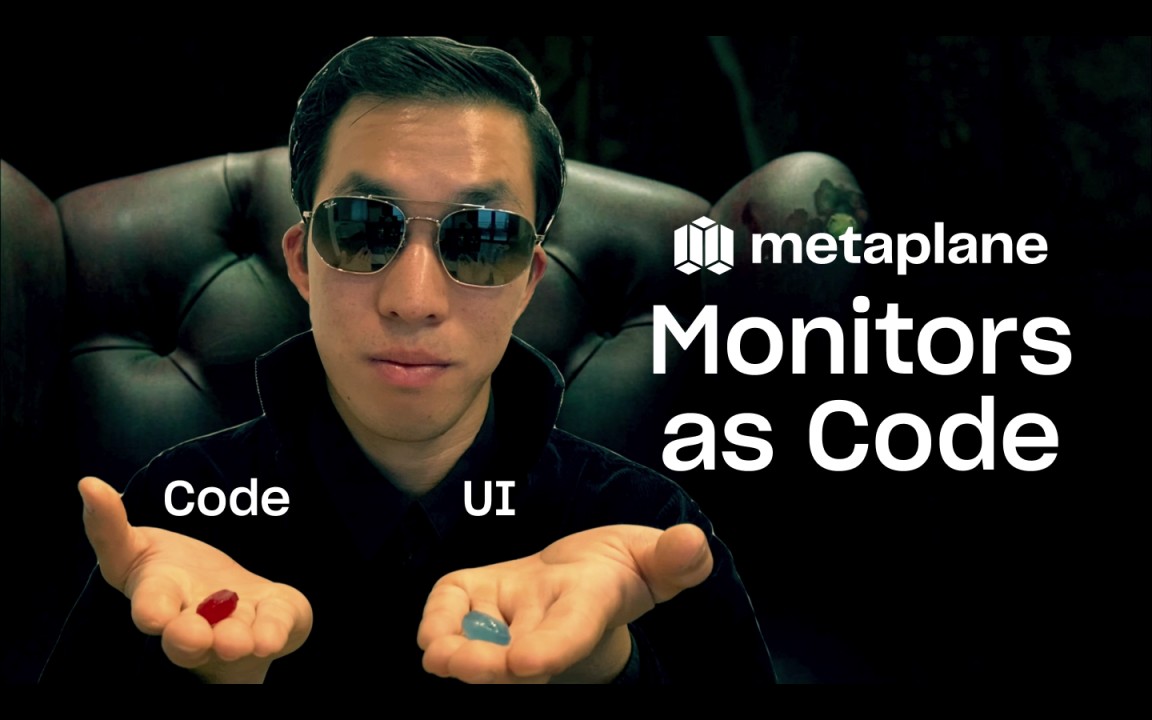

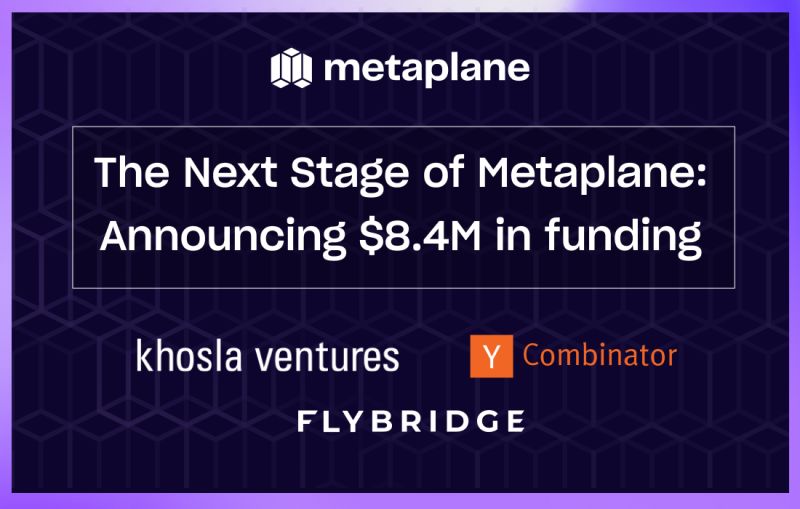
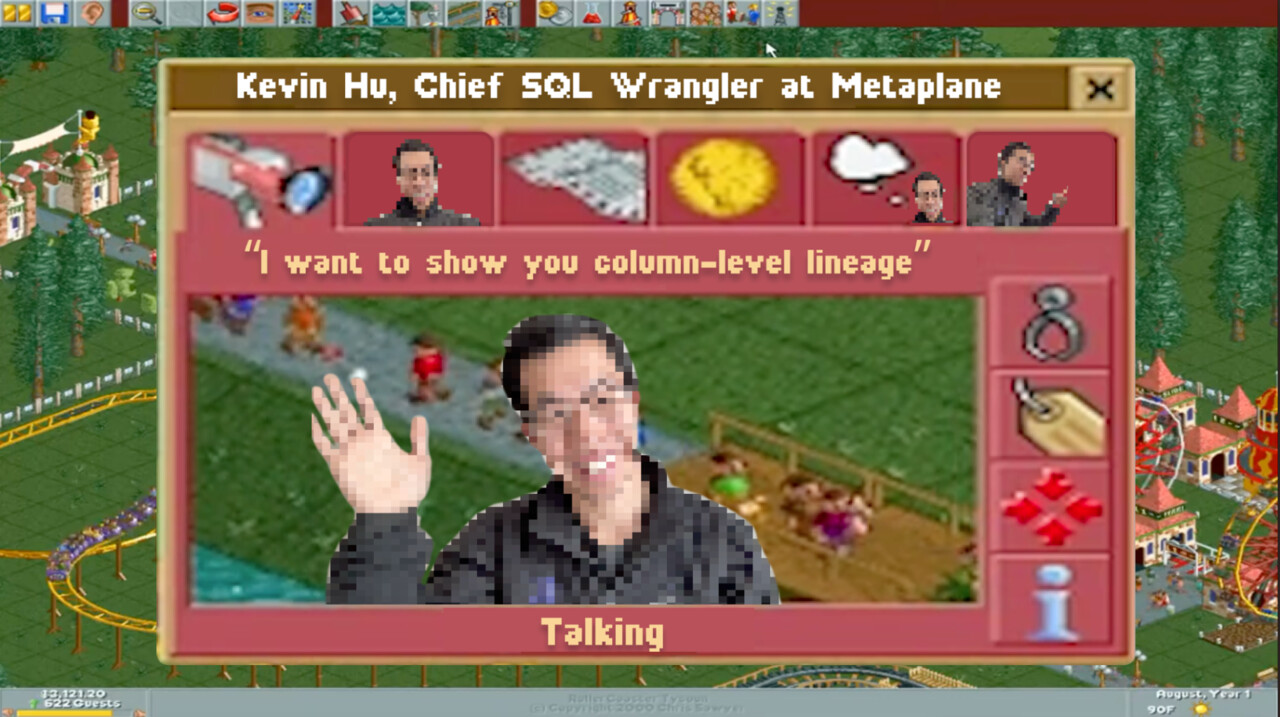
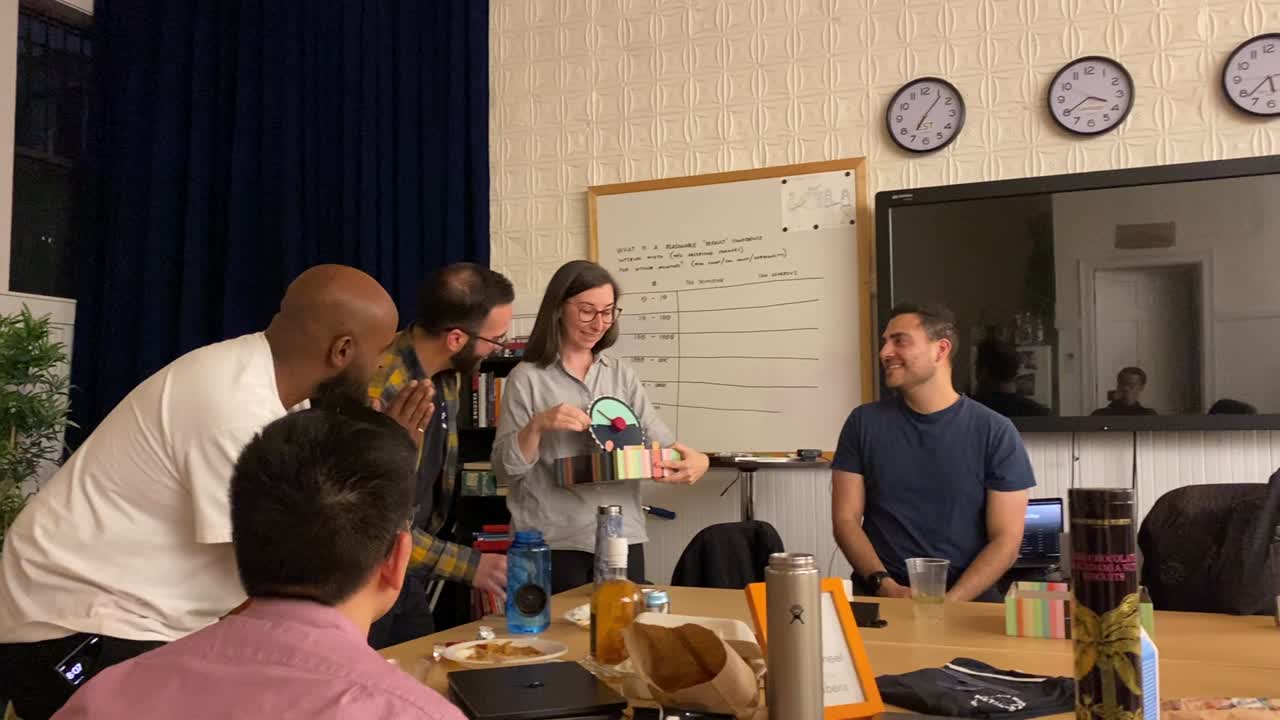